發布人:Google Research 研究員 Aurko Roy
開放域長格式問答 (LFQA) 是自然語言處理 (NLP) 的一項基礎挑戰,涉及檢索與給定問題相關的文檔,并使用這些文檔來生成一段詳盡答案。在事實型開放域問答 (QA) 中,簡單的短語或實體便足以回答問題。雖然我們近期在這一方面取得了顯著進展,但在長格式問答領域中卻做得遠遠不夠。盡管如此,LFQA 仍是一項非常重要的任務,特別是它能提供一個測試平臺來衡量生成文本模型的真實性。但是,當前的基準和評估指標真的能在 LFQA 方面取得進展嗎?
在“在長格式問答領域取得進展的障礙”(Hurdles to Progress in Long-form Question Answering)(將在 NAACL 2021 會議上發表)中,我們介紹了一種新的開放域長格式問答系統,它利用了 NLP 的兩項最新進展:
1.最先進的稀疏注意力模型(例如 Routing Transformer(RT)),能夠將基于注意力的模型擴展至長序列;
2.基于檢索的模型(例如 REALM),有助于檢索與給定查詢相關的維基百科文章。
Routing Transformer
https://www.mitpressjournals.org/doi/full/10.1162/tacl_a_00353
為獲得更多的事實依據,對于檢索到的與給定問題相關的一些維基百科文章,我們的系統會在答案生成之前將從中獲得的信息結合起來 ELI5 是唯一一個可用于長格式問答的大規模公開數據集,我們的系統在該數據集上取得了突破性進展。
ELI5
https://ai.facebook.com/blog/longform-qa/
不過,雖然這個系統在公開排行榜上名列前茅,但我們發現 ELI5 數據集及其相關評估指標的一些趨勢令人擔憂。特別要強調的是,我們發現 1) 幾乎沒有證據表明模型實際使用了它們所要求的檢索;2) 平凡基線(例如輸入復制)擊敗了現代系統,如 RAG/BART+DPR;以及 3) 數據集中存在大量訓練/驗證重疊。我們的論文針對每一個問題提出了緩解策略。
輸入復制
https://eval.ai/web/challenges/challenge-page/689/leaderboard/1908#leaderboardrank-6
文本生成
NLP 模型的核心要件是 Transformer 架構,其序列中的每個 Token 都會關注序列中的其他所有 Toekn,從而形成一個隨序列長度呈二次增長的模型。RT 模型引入了一種基于內容的動態稀疏注意力機制,將 Transformer 模型中的注意力復雜度從 n2 降到了 n1.5( 其中 n 是序列長度),使其能夠擴展到長序列。這使得每個單詞都可以關注整個文本中 任何地方的其他相關單詞, 而不像 Transformer XL 等類似方法,一個單詞只能關注其附近的單詞。
RT 發揮作用的關鍵在于每個 Token 對其他 Token 的關注通常是冗余的,并且可以通過結合局部和全局注意力進行估算。局部注意力允許每個 Token 在模型的幾個層上建立一個局部表征,其中每個 Token 關注一個局部鄰域,從而達到局部的一致性和流暢性。作為對局部注意力的補充,RT 模型還使用了小批量 k-均值集群, 使每個 Token 只關注一組最相關的 Token 。
我們以語言建模為目標,使用 ProjectGutenberg(PG-19) 數據集預先訓練了一個 RT 模型,即在給定前面所有單詞的情況下,讓該模型學會預測下一個單詞,從而能夠生成流利的段落長文本。
ProjectGutenberg(PG-19)
https://deepmind.com/blog/article/A_new_model_and_dataset_for_long-range_memory
信息檢索
為了證明 RT 模型在 LFQA 任務中的有效性,我們將其與 REALM 中檢索到的內容結合使用。REALM 模型(Guu 等人于 2020 年發布)是基于檢索的模型,使用最大內積搜索來檢索與特定查詢或問題相關的維基百科文章。我們對該模型進行了微調,以便根據自然問題數據集作出事實型問答。REALM 利用 BERT 模型學習問題的良好表征,并使用 SCANN 檢索與問題表征具有高度主題相似性的維基百科文章。接著進行端到端訓練,以最大程度地提高 QA 任務的對數似然值。
通過使用對比損失,我們進一步提高了 REALM 檢索的質量。其背后的想法是讓問題表征更靠近其基本事實答案,并與其他小批量答案有所不同。這樣可以確保,當系統使用此問題表征檢索相關項目時,會返回與基本事實答案“類似”的文章。我們稱這種檢索器為對比型-REALM 或 c-REALM。
對比損失
https://towardsdatascience.com/contrastive-loss-explaned-159f2d4a87ec
評估
我們使用 ELI5 數據集對該模型進行了長格式問答測試。ELI5 數據集是 KILT 基準的一部分, 也是唯一 公開可用的大規模 LFQA 數據集。KILT 基準使用準確率 (R-Prec) 衡量文本檢索質量,并使用 ROUGE-L 衡量文本生成質量。將這兩項得分相加即可得出一個 KILT R-L 得分,該得分決定了模型在排行榜上的排名。在 KILT 的 ELI5 數據集上,我們對預先訓練好的 RT 模型和 c-REALM 檢索進行了微調。
我們提交的內容在 ELI5 長格式問答 KILT 排行榜上名列前茅,綜合 KILT R-L 得分為 2.36。對比先前 BART + DPR 排行榜的排名(KILT R-L 得分為 1.9)有了提升,同時具有與排行榜上其他模型相似的參數數量。在文本生成質量方面,我們發現 Rouge-L 與 T5、BART + DPR 和 RAG 相比,得分分別提高了 4.11、5.78 和 9.14。
排行榜
https://eval.ai/web/challenges/challenge-page/689/leaderboard/1908
RT 模型生成的示例
Q1
Why are almost all boats white?
Boats are generally painted white for obvious reasons, as they are inexpensive and easy to clean. Ships are also often painted to provide a visible visual contrast to the sea. Boats also often have high visibility ports to allow for easy international trade.
In addition, many boats have an air-breathing function that makes them easier to spot and stay afloat, which is a good thing if you're a pirate or trying to get into some country's waters. Lastly, many ships are built in the last few decades, so they are not as exotic looking as they were thirty years ago.
And lastly, many ships are made out of materials that are easy to work with, and so many of them are painted white as a practical matter. There are many, many more reasons for why ships are white, but those are the ones I know of. I'm sure there are many other reasons, but those are the ones I know of.
Edit: I forgot to mention that the other answers about visibility and easy to see ports are also true. Ships are painted to appear to be larger, and more expensive. That makes them appear more impressive, and so they are more likely to be bought by people who want to show off their wealth and status.
Q2
What causes the trail behind jets at high altitude?
The same thing that causes the clouds you see at the start of a storm to form in the first place. The air pressure changes as the jet moves through the air, causing it to cool down and condense into water droplets. These droplets are in fact a lot bigger than the water droplets in the clouds, so when the plane passes through them, they can cause the air pressure to change, causing the water droplets to condense into clouds.
This is what you see when you look at a cloud formation from the ground. In addition to this, when the plane passes through the clouds, it also moves through the air, causing it to change its temperature, causing the water droplets to condense and form clouds.
These clouds are often quite large, and can block out the sun. This is what you see when you look at a contrail. Finally, the water vapor that forms at high altitudes is cooled by the air above, and as it cools, it condenses into clouds. This is what you see when you look at a cumulonimbus cloud. These clouds are even larger than the water droplets in the clouds, and they block out the sun.
Q3
How do shampoo and conditioner work?
The key ingredients in shampoo and conditioner are usually surfactants, they tend to attract oil from your skin. Shampoo will usually contain an anti-dandruff agent (dandruff is caused by fungi in your skin which cause dandruff). They also contain some moisturizing agents (moisturizers are things that stay in your skin longer and protect your scalp from cleaning),
And a lot of anti-dandruff (dandruff is a side effect of high doses of both surfactants and moisturizers) Conditioner will contain moisturizing agents, like a skin moisturizer. They tend to cause oils to be separated from your hair (e.g. wash your hair with conditioner instead of shampoo). They both have a foaming agent that makes them foam up (usually Hydrogen Peroxide). They also have a foaming agent that stops dirt from attaching to your hair. Some conditioner will also suppress the action of the shampoo (to help prevent dirt from attaching to your hair).
Q4
When glancing at a clock, why does the first second after glancing at it sometimes feel longer than the rest?
This is known as chronostasis. Your brain gets used to seeing the second hand move after the first, so it ignores the first one. If you were to look at the clock a lot, you'd see the second hand move a lot more than the first, and you'd be able to tell the difference between the second and the first.
The second hand will appear to move much slower than the first hand because your brain is used to seeing the second hand move a lot more than the first. If you were to go on a long trip, you'd get used to the second hand moving a lot more than the first, and you'd have no problem telling the difference between the second and the first hand.
It's kind of like how you don't notice your nose when you're driving, but if you look at it for a while, you start to notice it. Your brain is just getting used to seeing the nose move a lot more than the other, and it can't tell the difference between the two. It's kind of like that with your eyes.
Q5
Why do Britain and other English empire countries still bow to monarchs? What real purpose does the queen serve?
Depends what you define as "purpose". There is no specific purpose for the British monarch - rather, they are figureheads that serve a role. The role is to have a high degree of influence over a country, though the rules of the monarchy can somewhat vary from country to country, and the ceremony of "kissing the royal corpse" does not always involve kissing the monarch. Whether that's the only reason for the custom, or if it was the only reason,
I can't say, but that's the reasoning. When the U.K. was in full power, the monarch was a puppet of Parliament, and the powers of the monarch were transferred to the Prime Minister and thus to the Prime Minister's deputy, who then became the Prime Minister. Since then, Parliament has been able to vote on legislation that goes through the monarch,
although they may still act as the monarch's representative in negotiating treaties, which can have very very deep consequences. The Queen's role, as a representative of Britain, doesn't necessarily involve her formal approval of any of the laws or legislation that goes through Parliament, though.
Q6
What exactly is fire, in detail? How can light and heat come from something we can't really touch?
Fire is the chemical reaction of fuel (oxygen) with (what we call) impurities (ash, soot, oil etc). These impurities are created due to a number of things. If you were to study the chemical composition of a log (or a candle) and analyse it you would discover the chemical formula (A, C, and G) which is a simplified version of what actually happens. There is always fuel, and there is always the ash/soot. Wood is made up of mostly carbon and hydrogen and these are the fuels that create the fire. Hydrogen and oxygen are reactive, and they form water (H2O) and CO2 (CO2). If we split a log into smaller pieces, we start to see the process of combustion as it occurs. The carbon is burned, and with the explosion of CO, H2O and H2CO2, we get water and carbon dioxide (mostly). If we smash a log together, we start to see what happens when we also hit another log with another log...imagine smashing two sand castles together. We see how the sand castles and brick castles form and collapse at different rates...then it starts to burn. The smoke, ash and flames are the actual combustion of fuel.
Q7
What goes on in those tall tower buildings owned by major banks?
The actual buildings are not there for profit but for show. There are many real world historical buildings that have little to no effect other than being a symbol of an economic entity or symbol of an era or location, and nothing more. For example look at Sears, Sears didn't care what went on inside, it was all about the \_appearance\_ of its location, the prestige of the location, the facilities and so on. It didn't care about how long it took it to operate, it was about how much people would pay to go see it. Sears was a landmark as a cultural movement and other big companies followed suit, so if you want to see a building you've never seen before, you have to go see Sears, just like you have to see a Toyota Camry for Toyota Camry. They used to be all about building new factories, some of them if I recall, but now that they're bigger, that means that more factory jobs are coming to them. You've probably seen them in stores as stores where people buy and sell stuff, so there aren't that many places for them to come from. Instead, it's just for show, a symbol of rich people.
在 LFQA 領域取得進展的障礙
不過,雖然此處介紹的 RT 系統在公開排行榜中名列前茅,但對模型和 ELI5 數據庫的詳細分析仍揭示了一些令人擔憂的趨勢。
Train/Valid Overlap
Many held-out questions are paraphrased in the training set. Best answer to similar train questions gets 27.4 ROUGE-L.
Lack of Grounding
Conditioning answer generation on random documents instead of relevant ones does not measurably impact its factual correctness. Longer outputs get higher ROUGE-L.
我們發現,幾乎沒有任何證據表明模型會將其文本生成實際定位到檢索文檔中。與 Wikipedia 中的隨機檢索搭配使用的微調 RT 模型(例如,隨機檢索 + RT),幾乎與 c-REALM + RT 模型(24.2 與 24.4 ROUGE-L)表現得一樣好。在訓練、驗證和測試 ELI5 數據集時,我們還發現了很多的重疊(幾個問題相互解釋),因此可能不再需要檢索。KILT 基準會單獨衡量檢索和生成的質量,但不確定文本生成是否會在實際情況中使用檢索。
與 RAG 和 BART + DPR 相比,平凡基線會獲得更高的 Rouge-L 分數
此外,在使用 Rouge-L 指標和平凡無意義基線(如隨機訓練集答案和輸入復制)來評估文本生成質量的過程中,我們發現了一些問題,并導致 Rouge-L 分數相對較高(甚至超過了 BART + DPR 和 RAG)。
結論
我們為基于 Routing Transformers 和 REALM 的長格式問答推出了一個系統,該系統在關于 ELI5 的 KILT 排行榜中名列前茅。但是,詳細的分析揭示了存在的一些問題,即無法使用基準來顯示有意義的建模進展。我們希望社區共同合作,一起解決這些問題,以便研究人員向正確的高峰攀登,在這個充滿挑戰但十分重要的任務中取得有意義的進展。
致謝
Routing Transformer 是 Aurko Roy、Mohammad Saffar、Ashish Vaswani 和 David Grangier 等人進行團隊協作的結果。有關開放域長格式問答的后續工作是由 Kalpesh Krishna、Aurko Roy 和 Mohit Iyyer 協作完成的。我們要感謝 Vidhisha Balachandran、Niki Parmar 和 Ashish Vaswani 提供的多條實用意見,感謝 REALM 團隊 (Kenton Lee、Kelvin Guu、Ming-Wei Chang 和 Zora Tung) 在代碼庫方面提供的幫助以及多條實用意見,這些意見幫助我們進一步完善了實驗。
我們非常感謝 Tu Vu 針對 QQP 分類器提供的幫助,這些分類器用于在 ELI5 訓練集和測試集中檢測解釋。感謝 Jules Gagnon-Marchand 和 Sewon Min 對檢查 ROUGE-L 邊界提供的有用實驗建議。最后,感謝 Shufan Wang、Andrew Drozdov、Nader Akoury 以及 UMass NLP 小組的其他成員針對項目的不同階段提出的實用意見和建議。
編輯:jq
-
數據集
+關注
關注
4文章
1208瀏覽量
24719 -
nlp
+關注
關注
1文章
489瀏覽量
22049
原文標題:開放域長格式問答系統的進步與挑戰
文章出處:【微信號:tensorflowers,微信公眾號:Tensorflowers】歡迎添加關注!文章轉載請注明出處。
發布評論請先 登錄
相關推薦
混合域示波器的原理和應用
TE一站式解決方案,助您“域”見未來
50萬獎金池!開放原子大賽——第二屆OpenHarmony創新應用挑戰賽正式啟動
【?嵌入式機電一體化系統設計與實現?閱讀體驗】+磁力輸送機系統設計的創新與挑戰
浪潮信息趙帥:開放計算創新 應對Scaling Law挑戰
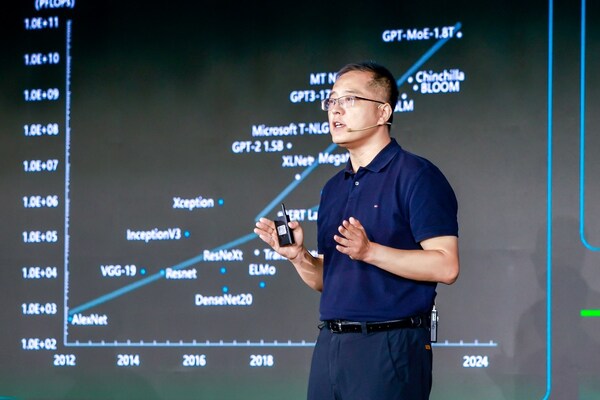
CAN數據幀的各個域及其作用
llm模型有哪些格式
請問CAN數據域的數據格式該如何定義?以什么標準定義?
開放原子開源大賽OpenHarmony智能化應用生態挑戰賽決賽路成功舉辦
什么是智能汽車第六域?
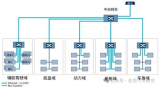
評論