隨著機器學習的發展,TinyML(微型機器學習)已在你的家里、車里、甚至口袋里工作了。什么是 TinyML 呢?它是屬于機器學習的一個子領域,包含了算法、硬件和軟件,能夠基于傳感器數據分析,并在極低功耗的設備上運行。比如家里的天貓精靈、蘋果的 Siri 和亞馬遜的 Alexa 等語音助手,都屬于 TinyML 的應用,它們提供了語音用戶接口 (AUI),讓用戶不需要操作屏幕、鼠標或鍵盤就可以進行交互,給用戶提供了一種全新的交互方式,這些語音助手幾乎無處不在。從獨立的智能音箱到幾乎所有手機都內置了某種語音助手。
在大部分情況下,語音識別、自然語言處理以及語音合成等繁重工作都是在云端完成的,由性能強大的服務器運行大型機器學習模型。當用戶提出問題時,將以 wav 或其他音頻流的形式被發送到云端。云端識別出音頻流的含義并進行回復響應。語音助手真正需要的音頻是喚醒設備后的數據。如果能在不發送數據的情況下檢測到這個喚醒詞,并在聽到喚醒詞之后才開始音頻流的傳輸,這樣既能夠保護用戶的隱私、節省電池電量和帶寬,而且可以在沒有網絡的情況下喚醒。
這也是 TinyML 的用武之地。要在低功耗的芯片上運行,意味著要訓練一個監聽喚醒詞的微型模型,嵌入到微控制設備中,它就可以一直監聽喚醒詞,當檢測到喚醒詞后通知操作系統開始捕獲音頻并發送到云端。
在本文章中,將教大家如何使用預先訓練的檢測模型,在 HaaS EDU K1 上進行喚醒詞檢測模型的部署,并使用 Tensorflow Lite Micro 推理引擎進行推理。隨后將教大家如何使用 Tensorflow 訓練腳本訓練一個自己的喚醒詞,再進行設備端部署。本案例主要由三個部分組成:
1. 語音采集:接入模擬麥克風(Mic1 輸入);
2. 語音識別:說出“打開”和“關閉”識別后,OLED 將顯示 “Turn on.。.” 和 “Turn off”;
3. 語音播報:執行指令的同時,播報本地 TTS (mp3)。
涉及知識點
●
喚醒詞數據采集、模型訓練、模型部署
●
設備端模擬 MIC 聲音采樣
●
設備端音頻特征提取
●
TFLite-Micro 推理引擎應用
●
設備端命令識別、響應
●
設備端喇叭播放 mp3 文件
●
文件系統應用
●
OLED 顯示字符
方案介紹
整個方案的框架如下:
●
提供批量音頻錄制工具進行數據收集;
●
提供 TF 模型訓練腳本進行喚醒詞訓練;
●
提供完整設備端模型部署方案;
基于該方案,你將學習到 TinyML 的整個生命周期:
開發環境搭建
1. 硬件準備
如果有 HaaS 語音擴展板,直接插入即可:
如果沒有 HaaS 語音擴展板,請按照如下步驟接入麥克風和喇叭:
HaaS EDU K1 硬件排線圖請參考
HaaS EDU K1 說明書 - IoT 物聯網操作系統 - 阿里云
HaaS EDU K1 說明書 - IoT 物聯網操作系統 - 阿里云
https://help.aliyun.com/document_detail/205267.html
2. 環境搭建
參考《HaaS EDU K1 快速開始》中 HaaS Studio 章節完成 AliOS Things 開發環境搭建。
《HaaS EDU K1 快速開始》
https://blog.csdn.net/HaaSTech/article/details/119674142
2.1 案例代碼下載
該案例相關的源代碼下載可參考《創建工程》,該案例是 C/C++ 案例。
《創建工程》
https://g.alicdn.com/alios-things-3.3/doc/haas-studio.html?spm=a2cti.24227744.0.0.696b40faTNQpKM#autotoc_md1867
其中:
●
選擇解決方案: “TFLite-Micro 離線語音快捷詞喚醒案例”或者 “tflite_micro_speech_demo”
●
選擇開發板: HaaS EDU K1
2.2 代碼編譯、燒錄
參考《HaaS EDU K1 快速開始》完成代碼的編譯及燒錄,在燒錄前,請先完成 2.1 的步驟,再進行編譯燒錄。
《HaaS EDU K1 快速開始》
https://blog.csdn.net/HaaSTech/article/details/119674142
文件件系統燒錄
本組件例子中使用到的本地語料存放在代碼中 hardware/chip/haas1000/prebuild/data/ 目錄下 mp3 目錄,除燒錄 tflite_micro_speech_demo image 外,需燒錄 littlefs 文件系統,請將 hardware/chip/haas1000/package.yaml 文件中以下代碼段的注釋打開后重新編譯:
program_data_files:
- filename: release/write_flash_tool/ota_bin/littlefs.bin
address: 0xB32000
2.3 打開串口
參考《HaaS EDU K1 快速開始》打開串口進行 LOG 查看。
《HaaS EDU K1 快速開始》
https://blog.csdn.net/HaaSTech/article/details/119674142
軟件架構
●
KWS Demo 應用程序: 主要打通實現 AI 語音引擎的初始化,歡迎語播報。
●
ai_agent 組件:是 AliOS Things 上的 AI 引擎核心模塊,后端接入不同的推理引擎,本案例中使用了 TFLite-Micro 推理引擎。
●
uVoice 組件:是 AliOS Things 上智能語音解決方案的核心組件,提供了本地音頻,URL 音頻,TTS 合成等基礎功能,音頻格式支持 mp3, m4a, wav, opus 等主流格式,本案例中使用它來進行本地 mp3 語料的響應播報。
●
A2SA 組件:是 AliOS Things 上音頻服務框架,兼容 ALSA 應用接口訪問,支持音頻硬件驅動抽象,多音頻驅動加載/卸載,VFS 接口支持等功能。
1. 代碼結構
├── cp_resources.py # 拷貝本地語料到/prebuild/data目錄,編譯進文件系統
├── main.c
├── maintask.c
├── Makefile
├── micro_speech # 語音識別程序
├── oled # OLED顯示程序
│ ├── oled.c
│ └── oled.h
├── package.yaml # 編譯系統配置文件
├── player # 播放器程序
│ ├── player.c
│ └── player.h
├── README.md
├── recorder # 錄音程序
│ ├── recorder.c
│ └── recorder.h
├── resources
│ └── mp3 # 本地mp3語料
├── SConstruct
2. 設備端工作流程
在 HaaS EDU K1 上的整個工作流程如下圖:
3. 程序主體
以下代碼是執行喚醒詞識別主體,setup 對 TFLite-Micro 模型推理引擎進行初始化,loop 中執行上圖中整個流程,從音頻采集到命令響應的全部流程在該函數中實現,詳細邏輯請參考代碼。
// The name of this function is important for Arduino compatibility.
void setup()
{
// tflite::InitializeTarget();
//RegisterDebugLogCallback(callback);
// Set up logging. Google style is to avoid globals or statics because of
// lifetime uncertainty, but since this has a trivial destructor it‘s okay.
// NOLINTNEXTLINE(runtime-global-variables)
static tflite::MicroErrorReporter micro_error_reporter;
error_reporter = μ_error_reporter;
// Map the model into a usable data structure. This doesn’t involve any
// copying or parsing, it‘s a very lightweight operation.
model = tflite::GetModel(g_model);
if (model-》version() != TFLITE_SCHEMA_VERSION)
{
TF_LITE_REPORT_ERROR(error_reporter,
“Model provided is schema version %d not equal ”
“to supported version %d.”,
model-》version(), TFLITE_SCHEMA_VERSION);
return;
}
// Pull in only the operation implementations we need.
// This relies on a complete list of all the ops needed by this graph.
// An easier approach is to just use the AllOpsResolver, but this will
// incur some penalty in code space for op implementations that are not
// needed by this graph.
//
// tflite::AllOpsResolver resolver;
// NOLINTNEXTLINE(runtime-global-variables)
static tflite::MicroMutableOpResolver《4》 micro_op_resolver(error_reporter);
if (micro_op_resolver.AddDepthwiseConv2D() != kTfLiteOk)
{
return;
}
if (micro_op_resolver.AddFullyConnected() != kTfLiteOk)
{
return;
}
if (micro_op_resolver.AddSoftmax() != kTfLiteOk)
{
return;
}
if (micro_op_resolver.AddReshape() != kTfLiteOk)
{
return;
}
// Build an interpreter to run the model with.
static tflite::MicroInterpreter static_interpreter(
model, micro_op_resolver, tensor_arena, kTensorArenaSize, error_reporter);
interpreter = &static_interpreter;
// Allocate memory from the tensor_arena for the model’s tensors.
TfLiteStatus allocate_status = interpreter-》AllocateTensors();
if (allocate_status != kTfLiteOk)
{
TF_LITE_REPORT_ERROR(error_reporter, “AllocateTensors() failed”);
return;
}
// Get information about the memory area to use for the model‘s input.
model_input = interpreter-》input(0);
if ((model_input-》dims-》size != 2) || (model_input-》dims-》data[0] != 1) ||
(model_input-》dims-》data[1] !=
(kFeatureSliceCount * kFeatureSliceSize)) ||
(model_input-》type != kTfLiteInt8))
{
TF_LITE_REPORT_ERROR(error_reporter,
“Bad input tensor parameters in model”);
return;
}
model_input_buffer = model_input-》data.int8;
// Prepare to access the audio spectrograms from a microphone or other source
// that will provide the inputs to the neural network.
// NOLINTNEXTLINE(runtime-global-variables)
static FeatureProvider static_feature_provider(kFeatureElementCount,
feature_buffer);
feature_provider = &static_feature_provider;
static RecognizeCommands static_recognizer(error_reporter);
recognizer = &static_recognizer;
previous_time = 0;
}
// The name of this function is important for Arduino compatibility.
void loop()
{
// Fetch the spectrogram for the current time.
const int32_t current_time = LatestAudioTimestamp();
int how_many_new_slices = 0;
TfLiteStatus feature_status = feature_provider-》PopulateFeatureData(
error_reporter, previous_time, current_time, &how_many_new_slices);
// LOG(“current_time: %d, previous_time: %d, how_many_new_slices: %d
”, current_time, previous_time, how_many_new_slices);
if (feature_status != kTfLiteOk)
{
TF_LITE_REPORT_ERROR(error_reporter, “Feature generation failed”);
return;
}
previous_time = current_time;
// If no new audio samples have been received since last time, don’t bother
// running the network model.
if (how_many_new_slices == 0)
{
//LOG(“[lk added]how_many_new_slices is 0
”);
return;
}
// Copy feature buffer to input tensor
for (int i = 0; i 《 kFeatureElementCount; i++)
{
model_input_buffer[i] = feature_buffer[i];
}
// Run the model on the spectrogram input and make sure it succeeds.
TfLiteStatus invoke_status = interpreter-》Invoke();
if (invoke_status != kTfLiteOk)
{
TF_LITE_REPORT_ERROR(error_reporter, “Invoke failed”);
return;
}
// Obtain a pointer to the output tensor
TfLiteTensor *output = interpreter-》output(0);
// Determine whether a command was recognized based on the output of inference
const char *found_command = nullptr;
uint8_t score = 0;
bool is_new_command = false;
TfLiteStatus process_status = recognizer-》ProcessLatestResults(
output, current_time, &found_command, &score, &is_new_command);
if (process_status != kTfLiteOk)
{
TF_LITE_REPORT_ERROR(error_reporter,
“RecognizeCommands::ProcessLatestResults() failed”);
return;
}
// Do something based on the recognized command. The default implementation
// just prints to the error console, but you should replace this with your
// own function for a real application.
RespondToCommand(error_reporter, current_time, found_command, score,
is_new_command);
}
案例體驗
當程序燒錄完成后,直接喊出“打開“/“關閉”,就可以看到視頻所示的效果。目前只支持近場喚醒,喚醒距離 1 米左右。由于這個“打開”/“關閉”喚醒的語料有限,喚醒效果因人不同有差異。建議按照章節 6 中自己訓練一個喚醒詞或者使用數據集中的英文語料 “on/off” 試試。
自訓練喚醒詞
本案例是自訓練了一個“打開”/“關閉”快捷喚醒詞。本小節將帶你訓練一個新的快捷喚醒詞。
從錄音采集到部署到 HaaS EDU K1 的整個詳細流程如下:
1. 語料采集
語料采集是一個比較耗費人力的事情,通常商業化工程中語料收集有專人或專門的數據公司收集整理,這里提供了一個使用 Python 寫的錄音工具,方便你快速錄音。
依賴項安裝
#pip install pyaudio或者#conda install pyaudio
錄音配置
●
語音文件長度一秒
●
單聲道、16KHz、wav 格式
●
快、中、慢三種不同速度進行錄制
●
錄制次數 100 次以上,次數越多效果越好
●
相對安靜環境
1.1 喚醒詞錄制
錄制時看到“開始錄音,請說話。..。..”即可立即說出喚醒詞,比如“打開”、“關閉”。由于我們檢測一秒的喚醒詞,所以注意要在一秒內說完整整個喚醒詞,錄制一次后會自動回放確認是否錄制完整,如果錄制完整,按回車鍵繼續下一次錄制,如果錄制不完整或有其他雜音,按其他任意鍵刪除剛才的錄音再繼續下一次錄制。
執行命令:
#python micro_speech/train/record.py
毫無疑問,這個教學案例是教你如何錄制一個人的聲音,如果想要達到商業化的識別率,就至少需要 500 人以上的聲音錄制。如果僅僅錄制你一個人的喚醒詞,那么僅識別你的聲音是可以的,但其他人在喚醒時的成功率就會低很多。這個案例重點是教你了解喚醒詞訓練部署的原理。
1.2 背景噪音錄制
為了更好的識別,需要錄制一些背景噪音,模型訓練時會學習喚醒詞和背景噪音的差別。背景噪音可以錄制 1~2 分鐘。模型訓練時會自動從中隨機選擇片段作為背噪加入喚醒詞中進行學習。
執行命令:
#python micro_speech/train/record_noise.py
錄制背景噪音,放到 dataset/_background_noise_ 目錄。
1.3 創建自己的數據集
訓練腳本中默認采樣的預訓練數據集是 Google 發布的 Speech Commands(語音命令)數據集,該數據集是英文數據集。這里我們以錄制中文的“打開”/“關閉”為例,每個詞錄制 100 次。錄制完成后分別命名為 dakai 和 guanbi 兩個文件夾放入自定義的 my_dataset 目錄,然后從 Speech Commands 中選擇幾個單詞 house、marvin、wow 等喚醒詞作為“未知”類別放入到 my_only_dataset 目錄,它的作用是模型訓練時能夠從這些喚醒詞中識別想要的 dakai 和 guanbi 命令,dakai 和 guanbi 可以理解為正面示例,“未知”類別為反面示例。整個命令詞個數盡量限制在十個以下,這樣訓練的時間不會過久。如果你有其他同樣長度且與錄音配置中格式一樣的喚醒詞,也可以加入進來。另外如果錄制的是 100 次喚醒詞,那么其他作為“未知”類別的喚醒詞的錄音示例個數也盡量在 100 左右。錄制的背景噪音放入到 _background_noise_ 目錄,訓練時腳本將自動從中隨機選取一秒片段作為背景噪音加入到“無聲”類別中。
2. 模型訓練
2.1 PC 端訓練
PC 上在 VSCode 中使用 jupyter notebook 插件打開 tflite_micro_speech_demo/micro_speech/train/train_micro_speech_model.ipynb
進行其他喚醒詞的訓練。
前提:
參考《HaaS AI 之 VSCode 中搭建 Python 虛擬環境》搭建完開發環境后,安裝 tensorflow 1.15 版本:
《HaaS AI 之 VSCode 中搭建 Python 虛擬環境》
https://f.cp46.cn/q5zd
#conda create --name tf python=3.6#conda activate tf#conda install tensorflow=1.15
2.2 阿里云 PAI 平臺訓練
如果 PC 性能有限,使用阿里云 PAI 平臺進行訓練也是一個不錯的選擇,PAI-DSW 是一款云端機器學習開發 IDE,為您提供交互式編程環境,適用于不同水平的開發者。你可以根據需要選擇個人版、GPU 特價版或探索者版(免費),相關使用手冊 DSW 新手使用手冊。
探索者版(免費)
https://dsw-dev.data.aliyun.com/#/
DSW 新手使用手冊
https://tianchi.aliyun.com/forum/postDetail?spm=TODO.TODO.header.2.21864825A5pdfB&postId=121050
以使用 DSW 個人版為例:
1. 登錄 PAI 控制臺。
登錄 PAI 控制臺
https://pai.data.aliyun.com/console
2. 在左側導航欄,選擇模型開發和訓練 》 交互式建模 (DSW)。
3. 在頁面左上方,選擇目標地域。
4. 在 Notebook 建模服務頁面,單擊創建實例。
5. 在配置實例向導頁面,配置參數,鏡像選擇 tensorflow1.15-gpu-py36-cu101-ubuntu18.04 版本。
2.3 模型配置
無論在什么平臺上進行訓練,腳本中需要對訓練的參數進行一定的配置:
喚醒詞配置
WANTED_WORDS 就是你訓練的喚醒詞。比如:
WANTED_WORDS=”yes, on“,yes/on 對應于數據集 dataset 目錄的喚醒詞語料文件夾。這里根據你要訓練的喚醒詞修改。
訓練步數配置
如果你的喚醒詞僅僅數百條甚至數 10 條,那么訓練的步數不用太久,修改:
TRANINGS_STEPS=”1200, 300“
如果你有上千條以上,訓練的步數可以增加。
TRANINGS_STEPS=”15000, 3000“
為了防止訓練欠擬合或者過擬合,訓練的時間長短需要反復驗證,找到最優的結果。
數據集配置
如果使用自己的數據集,請修改:
DATASET_DIR = ‘。/dataset/’
3. 模型部署
模型部署在 HaaS EDU K1 上,主要有三個步驟:
1. 模型替換:將生成的模型文件 model.cc 替換 micro_speech/micro_features/model.cc 文件
2. 標簽更新:在 micro_speech/micro_features/micro_model_settings.cc 中修改 kCategoryLabels 標簽內容,將標簽名更換為你訓練的快捷詞,比如“打開”、“關閉”。由于標簽與模型的輸出張量元素是按照順序進行匹配的,因此,需要按照將標簽提供給訓練腳本的順序列出這些標簽。
3. 業務邏輯更新:在 micro_speech/command_responder.cc 中根據標簽更新相應的業務邏輯。目前在聽到“打開”后,會打開 HaaS EDU K1 上 R/G/B LED 燈。你也可以修改邏輯比如通過 WiFi 打開遠程的風扇或燈。這里可以充分發揮你的想象力打造一些比較有意思的場景應用。
總結
本案例在 HaaS EDU K1 上基于 TFLite-Micro 推理引擎進行語音喚醒詞的部署。也提供了從喚醒詞采集到模型訓練、模型部署的全鏈路開發流程,幫助您深入理解在低功耗 MCU 上如何進行離線語音識別的開發部署,期待您打造更多屬于你的離線喚醒詞應用。
責任編輯:haq
-
物聯網
+關注
關注
2911文章
44849瀏覽量
375376 -
開發板
+關注
關注
25文章
5100瀏覽量
97856 -
機器學習
+關注
關注
66文章
8430瀏覽量
132858
原文標題:社區分享 | 基于TensorFlow Lite Micro在HaaS EDU K1物聯網開發板上玩轉TinyML之離線語音喚醒
文章出處:【微信號:tensorflowers,微信公眾號:Tensorflowers】歡迎添加關注!文章轉載請注明出處。
發布評論請先 登錄
相關推薦
【「基于大模型的RAG應用開發與優化」閱讀體驗】+大模型微調技術解讀
企業AI模型部署攻略
AI模型部署邊緣設備的奇妙之旅:目標檢測模型
在樹莓派上部署YOLOv5進行動物目標檢測的完整流程
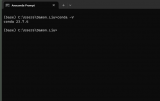
人臉檢測模型的精確度怎么算
人臉檢測模型有哪些
用OpenVINO C# API在intel平臺部署YOLOv10目標檢測模型
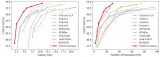
評論