發布人:TensorFlow 團隊的 Mathieu Guillame-Bert 和 Josh Gordon
隨機森林和梯度提升樹這類的決策森林模型通常是處理表格數據最有效的可用工具。與神經網絡相比,決策森林具有更多優勢,如配置過程更輕松、訓練速度更快等。使用樹可大幅減少準備數據集所需的代碼量,因為這些樹本身就可以處理數字、分類和缺失的特征。此外,這些樹通常還可提供開箱即用的良好結果,并具有可解釋的屬性。
盡管我們通常將 TensorFlow 視為訓練神經網絡的內容庫,但 Google 的一個常見用例是使用 TensorFlow 創建決策森林。
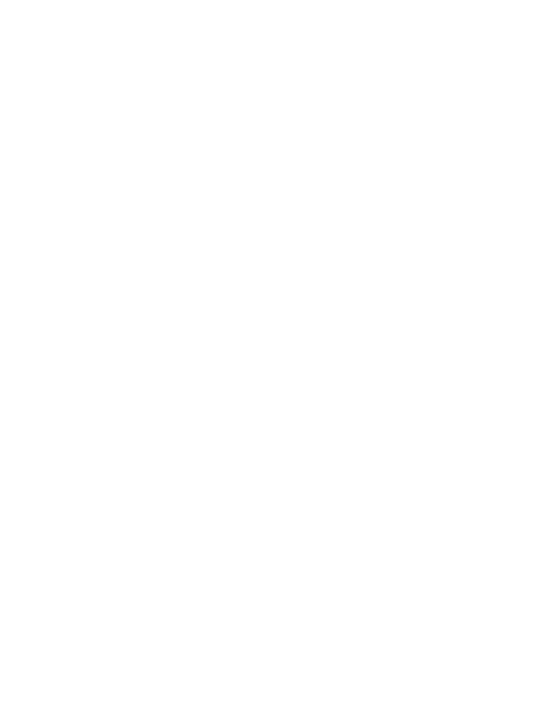
對數據開展分類的決策樹動畫
如果您曾使用 2019 年推出的 tf.estimator.BoostedTrees 創建基于樹的模型,您可參考本文所提供的指南進行遷移。雖然 Estimator API 基本可以應對在生產環境中使用模型的復雜性,包括分布式訓練和序列化,但是我們不建議您將其用于新代碼。
如果您要開始一個新項目,我們建議您使用 TensorFlow 決策森林 (TF-DF)。該內容庫可為訓練、服務和解讀決策森林模型提供最先進的算法,相較于先前的方法更具優勢,特別是在質量、速度和易用性方面表現尤為出色。
首先,讓我們來比較一下使用 Estimator API 和 TF-DF 創建提升樹模型的等效示例。
以下是使用 tf.estimator.BoostedTrees 訓練梯度提升樹模型的舊方法(不再推薦使用)
import tensorflow as tf
# Dataset generators
def make_dataset_fn(dataset_path):
def make_dataset():
data = ... # read dataset
return tf.data.Dataset.from_tensor_slices(...data...).repeat(10).batch(64)
return make_dataset
# List the possible values for the feature "f_2".
f_2_dictionary = ["NA", "red", "blue", "green"]
# The feature columns define the input features of the model.
feature_columns = [
tf.feature_column.numeric_column("f_1"),
tf.feature_column.indicator_column(
tf.feature_column.categorical_column_with_vocabulary_list("f_2",
f_2_dictionary,
# A special value "missing" is used to represent missing values.
default_value=0)
),
]
# Configure the estimator
estimator = boosted_trees.BoostedTreesClassifier(
n_trees=1000,
feature_columns=feature_columns,
n_classes=3,
# Rule of thumb proposed in the BoostedTreesClassifier documentation.
n_batches_per_layer=max(2, int(len(train_df) / 2 / FLAGS.batch_size)),
)
# Stop the training is the validation loss stop decreasing.
early_stopping_hook = early_stopping.stop_if_no_decrease_hook(
estimator,
metric_name="loss",
max_steps_without_decrease=100,
min_steps=50)
tf.estimator.train_and_evaluate(
estimator,
train_spec=tf.estimator.TrainSpec(
make_dataset_fn(train_path),
hooks=[
# Early stopping needs a CheckpointSaverHook.
tf.train.CheckpointSaverHook(
checkpoint_dir=input_config.raw.temp_dir, save_steps=500),
early_stopping_hook,
]),
eval_spec=tf.estimator.EvalSpec(make_dataset_fn(valid_path)))
使用 TensorFlow 決策森林訓練相同的模型
import tensorflow_decision_forests as tfdf
# Load the datasets
# This code is similar to the estimator.
def make_dataset(dataset_path):
data = ... # read dataset
return tf.data.Dataset.from_tensor_slices(...data...).batch(64)
train_dataset = make_dataset(train_path)
valid_dataset = make_dataset(valid_path)
# List the input features of the model.
features = [
tfdf.keras.FeatureUsage("f_1", keras.FeatureSemantic.NUMERICAL),
tfdf.keras.FeatureUsage("f_2", keras.FeatureSemantic.CATEGORICAL),
]
model = tfdf.keras.GradientBoostedTreesModel(
task = tfdf.keras.Task.CLASSIFICATION,
num_trees=1000,
features=features,
exclude_non_specified_features=True)
model.fit(train_dataset, valid_dataset)
# Export the model to a SavedModel.
model.save("project/model")
附注
-
雖然在此示例中沒有明確說明,但 TensorFlow 決策森林可自動啟用和配置早停。
-
可自動構建和優化“f_2”特征字典(例如,將稀有值合并到一個未登錄詞項目中)。
-
可從數據集中自動確定類別數(本例中為 3 個)。
-
批次大小(本例中為 64)對模型訓練沒有影響。以較大值為宜,因為這可以增加讀取數據集的效率。
TF-DF 的亮點就在于簡單易用,我們還可進一步簡化和完善上述示例,如下所示。
如何訓練 TensorFlow 決策森林(推薦解決方案)
import tensorflow_decision_forests as tfdf
import pandas as pd
# Pandas dataset can be used easily with pd_dataframe_to_tf_dataset.
train_df = pd.read_csv("project/train.csv")
# Convert the Pandas dataframe into a TensorFlow dataset.
train_ds = tfdf.keras.pd_dataframe_to_tf_dataset(train_df, label="my_label")
model = tfdf.keras.GradientBoostedTreeModel(num_trees=1000)
model.fit(train_dataset)
附注
-
我們未指定特征的語義(例如數字或分類)。在這種情況下,系統將自動推斷語義。
-
我們也沒有列出要使用的輸入特征。在這種情況下,系統將使用所有列(標簽除外)。可在訓練日志中查看輸入特征的列表和語義,或通過模型檢查器 API 查看。
-
我們沒有指定任何驗證數據集。每個算法都可以從訓練樣本中提取一個驗證數據集作為算法的最佳選擇。例如,默認情況下,如果未提供驗證數據集,則 GradientBoostedTreeModel 將使用 10% 的訓練數據進行驗證。
下面我們將介紹 Estimator API 和 TF-DF 的一些區別。
Estimator API 和 TF-DF 的區別
算法類型
TF-DF 是決策森林算法的集合,包括(但不限于)Estimator API 提供的梯度提升樹。請注意,TF-DF 還支持隨機森林(非常適用于干擾數據集)和 CART 實現(非常適用于解讀模型)。
此外,對于每個算法,TF-DF 都包含許多在文獻資料中發現并經過實驗驗證的變體 [1, 2, 3]。
精確與近似分塊的對比
TF1 GBT Estimator 是一種近似的樹學習算法。非正式情況下,Estimator 通過僅考慮樣本的隨機子集和每個步驟條件的隨機子集來構建樹。
默認情況下,TF-DF 是一種精確的樹訓練算法。非正式情況下,TF-DF 會考慮所有訓練樣本和每個步驟的所有可能分塊。這是一種更常見且通常表現更佳的解決方案。
雖然對于較大的數據集(具有百億數量級以上的“樣本和特征”數組)而言,有時 Estimator 的速度更快,但其近似值通常不太準確(因為需要種植更多樹才能達到相同的質量)。而對于小型數據集(所含的“樣本和特征”數組數目不足一億)而言,使用 Estimator 實現近似訓練形式的速度甚至可能比精確訓練更慢。
TF-DF 還支持不同類型的“近似”樹訓練。我們建議您使用精確訓練法,并選擇使用大型數據集測試近似訓練。
推理
Estimator 使用自上而下的樹路由算法運行模型推理。TF-DF 使用 QuickScorer 算法的擴展程序。
雖然兩種算法返回的結果完全相同,但自上而下的算法效率較低,因為這種算法的計算量會超出分支預測并導致緩存未命中。對于同一模型,TF-DF 的推理速度通常可提升 10 倍。
TF-DF 可為延遲關鍵應用程序提供 C++ API。其推理時間約為每核心每樣本 1 微秒。與 TF SavedModel 推理相比,這通常可將速度提升 50 至 1000 倍(對小型批次的效果更佳)。
多頭模型
Estimator 支持多頭模型(即輸出多種預測的模型)。目前,TF-DF 無法直接支持多頭模型,但是借助 Keras Functional API,TF-DF 可以將多個并行訓練的 TF-DF 模型組成一個多頭模型。
了解詳情
您可以訪問此網址,詳細了解 TensorFlow 決策森林。
如果您是首次接觸該內容庫,我們建議您從初學者示例開始。經驗豐富的 TensorFlow 用戶可以訪問此指南,詳細了解有關在 TensorFlow 中使用決策森林和神經網絡的區別要點,包括如何配置訓練流水線和關于數據集 I/O 的提示。
您還可以仔細閱讀從Estimator 遷移到 Keras API,了解如何從 Estimator 遷移到 Keras。
原文標題:如何從提升樹 Estimator 遷移到 TensorFlow 決策森林
文章出處:【微信公眾號:谷歌開發者】歡迎添加關注!文章轉載請注明出處。
-
Google
+關注
關注
5文章
1777瀏覽量
58382 -
模型
+關注
關注
1文章
3442瀏覽量
49663 -
tensorflow
+關注
關注
13文章
330瀏覽量
60843
原文標題:如何從提升樹 Estimator 遷移到 TensorFlow 決策森林
文章出處:【微信號:Google_Developers,微信公眾號:谷歌開發者】歡迎添加關注!文章轉載請注明出處。
發布評論請先 登錄
相關推薦
將TensorFlow模型轉換為中間表示 (IR) 時遇到不一致的形狀錯誤怎么解決?
將YOLOv4模型轉換為IR的說明,無法將模型轉換為TensorFlow2格式怎么解決?
為什么無法使用OpenVINO?模型優化器轉換TensorFlow 2.4模型?
為什么無法將自定義EfficientDet模型從TensorFlow 2轉換為中間表示(IR)?
宇樹科技在物聯網方面
快速部署Tensorflow和TFLITE模型在Jacinto7 Soc
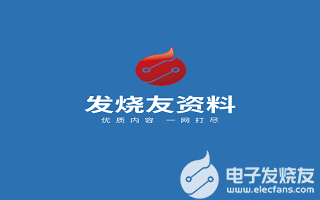
評論