在第 7 節中,我們研究了使用二維 CNN 處理二維圖像數據的機制,這些機制應用于相鄰像素等局部特征。盡管最初是為計算機視覺設計的,但 CNN 也廣泛用于自然語言處理。簡單地說,只需將任何文本序列視為一維圖像即可。通過這種方式,一維 CNN 可以處理局部特征,例如n- 文本中的克。
在本節中,我們將使用textCNN模型來演示如何設計用于表示單個文本的 CNN 架構 ( Kim, 2014 )。與圖 16.2.1使用帶有 GloVe 預訓練的 RNN 架構進行情感分析相比,圖 16.3.1的唯一區別在于架構的選擇。
圖 16.3.1本節將預訓練的 GloVe 提供給基于 CNN 的架構以進行情感分析。
import torch from torch import nn from d2l import torch as d2l batch_size = 64 train_iter, test_iter, vocab = d2l.load_data_imdb(batch_size)
from mxnet import gluon, init, np, npx from mxnet.gluon import nn from d2l import mxnet as d2l npx.set_np() batch_size = 64 train_iter, test_iter, vocab = d2l.load_data_imdb(batch_size)
16.3.1。一維卷積
在介紹模型之前,讓我們看看一維卷積是如何工作的。請記住,這只是基于互相關運算的二維卷積的特例。
圖 16.3.2一維互相關運算。陰影部分是第一個輸出元素以及用于輸出計算的輸入和核張量元素: 0×1+1×2=2.
如圖 16.3.2所示,在一維情況下,卷積窗口在輸入張量上從左向右滑動。在滑動過程中,輸入子張量(例如,0和1在 圖 16.3.2中)包含在某個位置的卷積窗口和內核張量(例如,1和2在 圖 16.3.2中)按元素相乘。這些乘法的總和給出單個標量值(例如, 0×1+1×2=2在圖 16.3.2中)在輸出張量的相應位置。
我們在以下函數中實現一維互相關 corr1d。給定一個輸入張量X和一個內核張量 K,它返回輸出張量Y。
def corr1d(X, K): w = K.shape[0] Y = torch.zeros((X.shape[0] - w + 1)) for i in range(Y.shape[0]): Y[i] = (X[i: i + w] * K).sum() return Y
def corr1d(X, K): w = K.shape[0] Y = np.zeros((X.shape[0] - w + 1)) for i in range(Y.shape[0]): Y[i] = (X[i: i + w] * K).sum() return Y
我們可以從 圖 16.3.2構造輸入張量X和核張量來驗證上述一維互相關實現的輸出。K
X, K = torch.tensor([0, 1, 2, 3, 4, 5, 6]), torch.tensor([1, 2]) corr1d(X, K)
tensor([ 2., 5., 8., 11., 14., 17.])
X, K = np.array([0, 1, 2, 3, 4, 5, 6]), np.array([1, 2]) corr1d(X, K)
array([ 2., 5., 8., 11., 14., 17.])
對于任何具有多個通道的一維輸入,卷積核需要具有相同數量的輸入通道。然后對于每個通道,對輸入的一維張量和卷積核的一維張量進行互相關運算,將所有通道的結果相加得到一維輸出張量。圖 16.3.3顯示了具有 3 個輸入通道的一維互相關運算。
圖 16.3.3具有 3 個輸入通道的一維互相關操作。陰影部分是第一個輸出元素以及用于輸出計算的輸入和核張量元素: 0×1+1×2+1×3+2×4+2×(?1)+3×(?3)=2.
我們可以對多個輸入通道進行一維互相關運算,并驗證 圖 16.3.3中的結果。
def corr1d_multi_in(X, K): # First, iterate through the 0th dimension (channel dimension) of `X` and # `K`. Then, add them together return sum(corr1d(x, k) for x, k in zip(X, K)) X = torch.tensor([[0, 1, 2, 3, 4, 5, 6], [1, 2, 3, 4, 5, 6, 7], [2, 3, 4, 5, 6, 7, 8]]) K = torch.tensor([[1, 2], [3, 4], [-1, -3]]) corr1d_multi_in(X, K)
tensor([ 2., 8., 14., 20., 26., 32.])
def corr1d_multi_in(X, K): # First, iterate through the 0th dimension (channel dimension) of `X` and # `K`. Then, add them together return sum(corr1d(x, k) for x, k in zip(X, K)) X = np.array([[0, 1, 2, 3, 4, 5, 6], [1, 2, 3, 4, 5, 6, 7], [2, 3, 4, 5, 6, 7, 8]]) K = np.array([[1, 2], [3, 4], [-1, -3]]) corr1d_multi_in(X, K)
array([ 2., 8., 14., 20., 26., 32.])
請注意,多輸入通道一維互相關等同于單輸入通道二維互相關。為了說明,圖 16.3.3中的多輸入通道一維互相關的等效形式是圖 16.3.4中的單輸入通道二維互相關 ,其中卷積核必須與輸入張量相同。
圖 16.3.4單輸入通道的二維互相關運算。陰影部分是第一個輸出元素以及用于輸出計算的輸入和核張量元素: 2×(?1)+3×(?3)+1×3+2×4+0×1+1×2=2.
圖 16.3.2和 圖 16.3.3中的輸出都只有一個通道。與第 7.4.2 節中描述的具有多個輸出通道的二維卷積相同 ,我們也可以為一維卷積指定多個輸出通道。
16.3.2。最大超時池化
同樣,我們可以使用池化從序列表示中提取最高值作為跨時間步長的最重要特征。textCNN 中使用的最大 隨時間池化與一維全局最大池化類似(Collobert等人,2011 年)。對于每個通道在不同時間步存儲值的多通道輸入,每個通道的輸出是該通道的最大值。請注意,max-over-time 池允許在不同的通道上使用不同數量的時間步長。
16.3.3。textCNN 模型
使用一維卷積和最大時間池化,textCNN 模型將單獨的預訓練標記表示作為輸入,然后為下游應用獲取和轉換序列表示。
對于單個文本序列n代表的代幣 d維向量,輸入張量的寬度、高度和通道數是n,1, 和d, 分別。textCNN 模型將輸入轉換為輸出,如下所示:
定義多個一維卷積核,分別對輸入進行卷積運算。具有不同寬度的卷積核可以捕獲不同數量的相鄰標記之間的局部特征。
對所有輸出通道執行 max-over-time 池化,然后將所有標量池化輸出連接為一個向量。
使用全連接層將串聯向量轉換為輸出類別。Dropout 可用于減少過度擬合。
圖 16.3.5 textCNN 的模型架構。
圖 16.3.5用一個具體的例子說明了 textCNN 的模型架構。輸入是一個包含 11 個標記的句子,其中每個標記由一個 6 維向量表示。所以我們有一個寬度為 11 的 6 通道輸入。定義兩個寬度為 2 和 4 的一維卷積核,分別具有 4 和 5 個輸出通道。它們產生 4 個寬度為11?2+1=10和 5 個寬度輸出通道11?4+1=8. 盡管這 9 個通道的寬度不同,但 max-over-time 池化給出了一個串聯的 9 維向量,最終將其轉換為用于二進制情感預測的 2 維輸出向量。
16.3.3.1。定義模型
我們在下面的類中實現了 textCNN 模型。與16.2節中的雙向RNN模型相比,除了用卷積層代替循環層外,我們還使用了兩個嵌入層:一個具有可訓練的權重,另一個具有固定的權重。
class TextCNN(nn.Module): def __init__(self, vocab_size, embed_size, kernel_sizes, num_channels, **kwargs): super(TextCNN, self).__init__(**kwargs) self.embedding = nn.Embedding(vocab_size, embed_size) # The embedding layer not to be trained self.constant_embedding = nn.Embedding(vocab_size, embed_size) self.dropout = nn.Dropout(0.5) self.decoder = nn.Linear(sum(num_channels), 2) # The max-over-time pooling layer has no parameters, so this instance # can be shared self.pool = nn.AdaptiveAvgPool1d(1) self.relu = nn.ReLU() # Create multiple one-dimensional convolutional layers self.convs = nn.ModuleList() for c, k in zip(num_channels, kernel_sizes): self.convs.append(nn.Conv1d(2 * embed_size, c, k)) def forward(self, inputs): # Concatenate two embedding layer outputs with shape (batch size, no. # of tokens, token vector dimension) along vectors embeddings = torch.cat(( self.embedding(inputs), self.constant_embedding(inputs)), dim=2) # Per the input format of one-dimensional convolutional layers, # rearrange the tensor so that the second dimension stores channels embeddings = embeddings.permute(0, 2, 1) # For each one-dimensional convolutional layer, after max-over-time # pooling, a tensor of shape (batch size, no. of channels, 1) is # obtained. Remove the last dimension and concatenate along channels encoding = torch.cat([ torch.squeeze(self.relu(self.pool(conv(embeddings))), dim=-1) for conv in self.convs], dim=1) outputs = self.decoder(self.dropout(encoding)) return outputs
class TextCNN(nn.Block): def __init__(self, vocab_size, embed_size, kernel_sizes, num_channels, **kwargs): super(TextCNN, self).__init__(**kwargs) self.embedding = nn.Embedding(vocab_size, embed_size) # The embedding layer not to be trained self.constant_embedding = nn.Embedding(vocab_size, embed_size) self.dropout = nn.Dropout(0.5) self.decoder = nn.Dense(2) # The max-over-time pooling layer has no parameters, so this instance # can be shared self.pool = nn.GlobalMaxPool1D() # Create multiple one-dimensional convolutional layers self.convs = nn.Sequential() for c, k in zip(num_channels, kernel_sizes): self.convs.add(nn.Conv1D(c, k, activation='relu')) def forward(self, inputs): # Concatenate two embedding layer outputs with shape (batch size, no. # of tokens, token vector dimension) along vectors embeddings = np.concatenate(( self.embedding(inputs), self.constant_embedding(inputs)), axis=2) # Per the input format of one-dimensional convolutional layers, # rearrange the tensor so that the second dimension stores channels embeddings = embeddings.transpose(0, 2, 1) # For each one-dimensional convolutional layer, after max-over-time # pooling, a tensor of shape (batch size, no. of channels, 1) is # obtained. Remove the last dimension and concatenate along channels encoding = np.concatenate([ np.squeeze(self.pool(conv(embeddings)), axis=-1) for conv in self.convs], axis=1) outputs = self.decoder(self.dropout(encoding)) return outputs
讓我們創建一個 textCNN 實例。它有 3 個卷積層,內核寬度分別為 3、4 和 5,都有 100 個輸出通道。
embed_size, kernel_sizes, nums_channels = 100, [3, 4, 5], [100, 100, 100] devices = d2l.try_all_gpus() net = TextCNN(len(vocab), embed_size, kernel_sizes, nums_channels) def init_weights(module): if type(module) in (nn.Linear, nn.Conv1d): nn.init.xavier_uniform_(module.weight) net.apply(init_weights);
embed_size, kernel_sizes, nums_channels = 100, [3, 4, 5], [100, 100, 100] devices = d2l.try_all_gpus() net = TextCNN(len(vocab), embed_size, kernel_sizes, nums_channels) net.initialize(init.Xavier(), ctx=devices)
16.3.3.2。加載預訓練詞向量
與第 16.2 節相同,我們加載預訓練的 100 維 GloVe 嵌入作為初始化的標記表示。這些令牌表示(嵌入權重)將在 中進行訓練embedding和固定constant_embedding。
glove_embedding = d2l.TokenEmbedding('glove.6b.100d') embeds = glove_embedding[vocab.idx_to_token] net.embedding.weight.data.copy_(embeds) net.constant_embedding.weight.data.copy_(embeds) net.constant_embedding.weight.requires_grad = False
glove_embedding = d2l.TokenEmbedding('glove.6b.100d') embeds = glove_embedding[vocab.idx_to_token] net.embedding.weight.set_data(embeds) net.constant_embedding.weight.set_data(embeds) net.constant_embedding.collect_params().setattr('grad_req', 'null')
16.3.3.3。訓練和評估模型
現在我們可以訓練用于情感分析的 textCNN 模型。
lr, num_epochs = 0.001, 5 trainer = torch.optim.Adam(net.parameters(), lr=lr) loss = nn.CrossEntropyLoss(reduction="none") d2l.train_ch13(net, train_iter, test_iter, loss, trainer, num_epochs, devices)
loss 0.067, train acc 0.978, test acc 0.869 2827.9 examples/sec on [device(type='cuda', index=0), device(type='cuda', index=1)]
lr, num_epochs = 0.001, 5 trainer = gluon.Trainer(net.collect_params(), 'adam', {'learning_rate': lr}) loss = gluon.loss.SoftmaxCrossEntropyLoss() d2l.train_ch13(net, train_iter, test_iter, loss, trainer, num_epochs, devices)
loss 0.089, train acc 0.970, test acc 0.867 1527.8 examples/sec on [gpu(0), gpu(1)]
下面我們使用經過訓練的模型來預測兩個簡單句子的情緒。
d2l.predict_sentiment(net, vocab, 'this movie is so great')
'positive'
d2l.predict_sentiment(net, vocab, 'this movie is so bad')
'negative'
d2l.predict_sentiment(net, vocab, 'this movie is so great')
'positive'
d2l.predict_sentiment(net, vocab, 'this movie is so bad')
'negative'
16.3.4。概括
一維 CNN 可以處理局部特征,例如 n- 文本中的克。
多輸入通道一維互相關等價于單輸入通道二維互相關。
max-over-time 池允許在不同的通道上使用不同數量的時間步長。
textCNN 模型使用一維卷積層和 max-over-time 池化層將單個標記表示轉換為下游應用程序輸出。
16.3.5。練習
調整超參數并比較16.2 節和本節中用于情感分析的兩種架構,例如分類精度和計算效率。
你能否利用16.2節習題中介紹的方法進一步提高模型的分類準確率 ?
在輸入表示中添加位置編碼。它會提高分類精度嗎?
-
神經網絡
+關注
關注
42文章
4771瀏覽量
100719 -
卷積
+關注
關注
0文章
95瀏覽量
18507 -
pytorch
+關注
關注
2文章
808瀏覽量
13201
發布評論請先 登錄
相關推薦
使用PyTorch深度解析卷積神經網絡
卷積神經網絡如何使用
卷積神經網絡模型發展及應用
基于深度學習的卷積神經網絡模型分析文本的情感傾向
PyTorch教程8.1之深度卷積神經網絡(AlexNet)
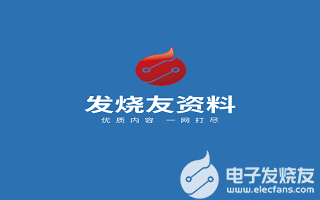
評論