在我們深入研究該方法之前,我們將首先討論一個基本的代碼結構,它使我們能夠有效地實現各種 HPO 算法。一般來說,這里考慮的所有 HPO 算法都需要實現兩個決策原語,即搜索和調度。首先,他們需要對新的超參數配置進行采樣,這通常涉及對配置空間的某種搜索。其次,對于每個配置,HPO 算法需要安排其評估并決定為其分配多少資源。一旦我們開始評估配置,我們就會將其稱為試用。我們將這些決定映射到兩個類,HPOSearcher和 HPOScheduler。除此之外,我們還提供HPOTuner執行優化過程的類。
這種調度器和搜索器的概念也在流行的 HPO 庫中實現,例如 Syne Tune (Salinas等人,2022 年)、Ray Tune (Liaw等人,2018 年)或 Optuna (Akiba等人,2019 年)。
import time from scipy import stats from d2l import torch as d2l
19.2.1。搜尋器
下面我們定義一個搜索器的基類,通過函數提供一個新的候選配置sample_configuration。實現此功能的一種簡單方法是隨機對配置進行統一采樣,就像我們在 第 19.1 節中對隨機搜索所做的那樣。更復雜的算法,例如貝葉斯優化,將根據先前試驗的表現做出這些決定。因此,隨著時間的推移,這些算法能夠對更有希望的候選人進行抽樣。我們添加該update 功能是為了更新以前試驗的歷史,然后可以利用它來改進我們的抽樣分布。
class HPOSearcher(d2l.HyperParameters): #@save def sample_configuration() -> dict: raise NotImplementedError def update(self, config: dict, error: float, additional_info=None): pass
以下代碼顯示了如何在此 API 中實現我們上一節中的隨機搜索優化器。作為一個輕微的擴展,我們允許用戶通過 指定要評估的第一個配置 initial_config,而隨后的配置是隨機抽取的。
class RandomSearcher(HPOSearcher): #@save def __init__(self, config_space: dict, initial_config=None): self.save_hyperparameters() def sample_configuration(self) -> dict: if self.initial_config is not None: result = self.initial_config self.initial_config = None else: result = { name: domain.rvs() for name, domain in self.config_space.items() } return result
19.2.2。調度程序
除了新試驗的采樣配置外,我們還需要決定何時進行試驗以及進行多長時間。實際上,所有這些決定都是由 完成的HPOScheduler,它將新配置的選擇委托給HPOSearcher. suggest只要某些訓練資源可用,就會調用該方法。除了調用sample_configuration搜索器之外,它還可以決定諸如max_epochs(即訓練模型的時間)之類的參數。update每當試驗返回新觀察時調用該方法。
class HPOScheduler(d2l.HyperParameters): #@save def suggest(self) -> dict: raise NotImplementedError def update(self, config: dict, error: float, info=None): raise NotImplementedError
要實現隨機搜索以及其他 HPO 算法,我們只需要一個基本的調度程序,它可以在每次新資源可用時調度新的配置。
class BasicScheduler(HPOScheduler): #@save def __init__(self, searcher: HPOSearcher): self.save_hyperparameters() def suggest(self) -> dict: return self.searcher.sample_configuration() def update(self, config: dict, error: float, info=None): self.searcher.update(config, error, additional_info=info)
19.2.3。調諧器
最后,我們需要一個組件來運行調度器/搜索器并對結果進行一些簿記。下面的代碼實現了 HPO 試驗的順序執行,在下一個訓練作業之后評估一個訓練作業,并將作為一個基本示例。我們稍后將使用 Syne Tune來處理更具可擴展性的分布式 HPO 案例。
class HPOTuner(d2l.HyperParameters): #@save def __init__(self, scheduler: HPOScheduler, objective: callable): self.save_hyperparameters() # Bookeeping results for plotting self.incumbent = None self.incumbent_error = None self.incumbent_trajectory = [] self.cumulative_runtime = [] self.current_runtime = 0 self.records = [] def run(self, number_of_trials): for i in range(number_of_trials): start_time = time.time() config = self.scheduler.suggest() print(f"Trial {i}: config = {config}") error = self.objective(**config) error = float(error.cpu().detach().numpy()) self.scheduler.update(config, error) runtime = time.time() - start_time self.bookkeeping(config, error, runtime) print(f" error = {error}, runtime = {runtime}")
19.2.4。簿記 HPO 算法的性能
對于任何 HPO 算法,我們最感興趣的是性能最佳的配置(稱為incumbent)及其在給定掛鐘時間后的驗證錯誤。這就是我們跟蹤runtime每次迭代的原因,其中包括運行評估的時間(調用 objective)和做出決策的時間(調用 scheduler.suggest)。在續集中,我們將繪制 cumulative_runtimeagainstincumbent_trajectory以可視化根據( 和) 定義的 HPO 算法的任何時間性能。這使我們不僅可以量化優化器找到的配置的工作情況,還可以量化優化器找到它的速度。schedulersearcher
@d2l.add_to_class(HPOTuner) #@save def bookkeeping(self, config: dict, error: float, runtime: float): self.records.append({"config": config, "error": error, "runtime": runtime}) # Check if the last hyperparameter configuration performs better # than the incumbent if self.incumbent is None or self.incumbent_error > error: self.incumbent = config self.incumbent_error = error # Add current best observed performance to the optimization trajectory self.incumbent_trajectory.append(self.incumbent_error) # Update runtime self.current_runtime += runtime self.cumulative_runtime.append(self.current_runtime)
19.2.5。示例:優化卷積神經網絡的超參數
我們現在使用隨機搜索的新實現來優化 第 7.6 節中卷積神經網絡的批量大小和學習率。我們通過定義目標函數,這將再次成為驗證錯誤。LeNet
def hpo_objective_lenet(learning_rate, batch_size, max_epochs=10): #@save model = d2l.LeNet(lr=learning_rate, num_classes=10) trainer = d2l.HPOTrainer(max_epochs=max_epochs, num_gpus=1) data = d2l.FashionMNIST(batch_size=batch_size) model.apply_init([next(iter(data.get_dataloader(True)))[0]], d2l.init_cnn) trainer.fit(model=model, data=data) validation_error = trainer.validation_error() return validation_error
我們還需要定義配置空間。此外,要評估的第一個配置是 第 7.6 節中使用的默認設置。
config_space = { "learning_rate": stats.loguniform(1e-2, 1), "batch_size": stats.randint(32, 256), } initial_config = { "learning_rate": 0.1, "batch_size": 128, }
現在我們可以開始隨機搜索了:
searcher = RandomSearcher(config_space, initial_config=initial_config) scheduler = BasicScheduler(searcher=searcher) tuner = HPOTuner(scheduler=scheduler, objective=hpo_objective_lenet) tuner.run(number_of_trials=5)
error = 0.17130666971206665, runtime = 125.33143877983093
下面我們繪制了現任者的優化軌跡,以獲得隨機搜索的任何時間性能:
board = d2l.ProgressBoard(xlabel="time", ylabel="error") for time_stamp, error in zip( tuner.cumulative_runtime, tuner.incumbent_trajectory ): board.draw(time_stamp, error, "random search", every_n=1)
19.2.6. 比較 HPO 算法
正如訓練算法或模型架構一樣,了解如何最好地比較不同的 HPO 算法非常重要。每次 HPO 運行取決于隨機性的兩個主要來源:訓練過程的隨機效應,例如隨機權重初始化或小批量排序,以及 HPO 算法本身的內在隨機性,例如隨機搜索的隨機抽樣。因此,在比較不同的算法時,至關重要的是多次運行每個實驗并報告基于隨機數生成器的不同種子的算法多次重復的總體統計數據,例如平均值或中值。
為了說明這一點,我們比較隨機搜索(參見第 19.1.2 節)和貝葉斯優化(Snoek等人,2012 年)在調整前饋神經網絡的超參數方面的作用。每個算法都經過評估50次使用不同的隨機種子。實線表示現任者在這些方面的平均表現 50重復和虛線標準偏差。我們可以看到隨機搜索和貝葉斯優化在大約 1000 秒內的表現大致相同,但貝葉斯優化可以利用過去的觀察來識別更好的配置,從而在之后迅速超越隨機搜索。
圖 19.2.1示例任意時間性能圖來比較兩種算法 A 和 B。
19.2.7。概括
本節列出了一個簡單而靈活的接口來實現我們將在本章中看到的各種 HPO 算法。在流行的開源 HPO 框架中可以找到類似的接口。我們還研究了如何比較 HPO 算法,以及需要注意的潛在陷阱。
19.2.8。練習
本練習的目標是為一個更具挑戰性的 HPO 問題實現目標函數,并運行更真實的實驗。我們將使用第 5.6 節DropoutMLP 中實現的兩個隱藏層 MLP 。
編寫目標函數,它應該取決于模型的所有超參數和batch_size。使用 max_epochs=50。GPU 在這里無濟于事,所以num_gpus=0. 提示:修改hpo_objective_lenet.
選擇一個合理的搜索空間,其中num_hiddens_1, num_hiddens_2是整數[8,1024], dropout 值位于[0,0.95], 而batch_size在于 [16,384]. 為 提供代碼config_space,使用來自 的合理分布scipy.stats。
對此示例運行隨機搜索number_of_trials=20并繪制結果。確保首先評估第 5.6 節的默認配置,即 .initial_config = {'num_hiddens_1': 256, 'num_hiddens_2': 256, 'dropout_1': 0.5, 'dropout_2': 0.5, 'lr': 0.1, 'batch_size': 256}
在本練習中,您將實現一個新的搜索器( 的子類 HPOSearcher),它根據過去的數據做出決策。這取決于參數probab_local, num_init_random。它的 sample_configuration工作原理如下。對于第一次 num_init_random調用,執行與 相同的操作 RandomSearcher.sample_configuration。否則,以概率 ,執行與 相同的操作 。否則,選擇迄今為止達到最小驗證錯誤的配置,隨機選擇其超參數之一,并像中一樣隨機采樣其值,但保持所有其他值相同。返回此配置,除了這個超參數外,它與迄今為止的最佳配置相同。1 - probab_localRandomSearcher.sample_configurationRandomSearcher.sample_configuration
編寫這個新的LocalSearcher. 提示:您的搜索者需要 config_space作為構造參數。隨意使用 type 的成員RandomSearcher。您還必須實施該update方法。
重新運行上一個練習中的實驗,但使用新的搜索器而不是RandomSearcher. 對,嘗試不同的值probab_local。num_init_random但是,請注意,不同 HPO 方法之間的適當比較需要多次重復實驗,并且理想情況下要考慮許多基準任務。
Discussions
-
算法
+關注
關注
23文章
4628瀏覽量
93186 -
參數
+關注
關注
11文章
1858瀏覽量
32375 -
pytorch
+關注
關注
2文章
808瀏覽量
13322
發布評論請先 登錄
相關推薦
Pytorch模型訓練實用PDF教程【中文】
改善深層神經網絡--超參數優化、batch正則化和程序框架 學習總結
如何利用PyTorch API構建CNN?
PerferredNetworks發布了其超參數優化框架的beta版本
自訓練Pytorch模型使用OpenVINO?優化并部署在AI愛克斯開發板
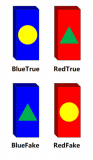
評論