1、引言
在CTR預(yù)估中,為了解決稀疏特征的問題,學(xué)者們提出了FM模型來建模特征之間的交互關(guān)系。但是FM模型只能表達(dá)特征之間兩兩組合之間的關(guān)系,無法建模兩個(gè)特征之間深層次的關(guān)系或者說多個(gè)特征之間的交互關(guān)系,因此學(xué)者們通過Deep Network來建模更高階的特征之間的關(guān)系。
因此 FM和深度網(wǎng)絡(luò)DNN的結(jié)合也就成為了CTR預(yù)估問題中主流的方法。有關(guān)FM和DNN的結(jié)合有兩種主流的方法,并行結(jié)構(gòu)和串行結(jié)構(gòu)。兩種結(jié)構(gòu)的理解以及實(shí)現(xiàn)如下表所示:
今天介紹的NFM模型(Neural Factorization Machine),便是串行結(jié)構(gòu)中一種較為簡單的網(wǎng)絡(luò)模型。
2、NFM模型介紹
我們首先來回顧一下FM模型,F(xiàn)M模型用n個(gè)隱變量來刻畫特征之間的交互關(guān)系。這里要強(qiáng)調(diào)的一點(diǎn)是,n是特征的總數(shù),是one-hot展開之后的,比如有三組特征,兩個(gè)連續(xù)特征,一個(gè)離散特征有5個(gè)取值,那么n=7而不是n=3.
順便回顧一下化簡過程:
可以看到,不考慮最外層的求和,我們可以得到一個(gè)K維的向量。
對(duì)于NFM模型,目標(biāo)值的預(yù)測公式變?yōu)椋?/p>
其中,f(x)是用來建模特征之間交互關(guān)系的多層前饋神經(jīng)網(wǎng)絡(luò)模塊,架構(gòu)圖如下所示:
Embedding Layer和我們之間幾個(gè)網(wǎng)絡(luò)是一樣的,embedding 得到的vector其實(shí)就是我們在FM中要學(xué)習(xí)的隱變量v。
Bi-Interaction Layer名字挺高大上的,其實(shí)它就是計(jì)算FM中的二次項(xiàng)的過程,因此得到的向量維度就是我們的Embedding的維度。最終的結(jié)果是:
Hidden Layers就是我們的DNN部分,將Bi-Interaction Layer得到的結(jié)果接入多層的神經(jīng)網(wǎng)絡(luò)進(jìn)行訓(xùn)練,從而捕捉到特征之間復(fù)雜的非線性關(guān)系。
在進(jìn)行多層訓(xùn)練之后,將最后一層的輸出求和同時(shí)加上一次項(xiàng)和偏置項(xiàng),就得到了我們的預(yù)測輸出:
是不是很簡單呢,哈哈。
3、代碼實(shí)戰(zhàn)
終于到了激動(dòng)人心的代碼實(shí)戰(zhàn)環(huán)節(jié)了,本文的代碼有不對(duì)的的地方或者改進(jìn)之處還望大家多多指正。
本文的github地址為:https://github.com/princewen/tensorflow_practice/tree/master/recommendation/Basic-NFM-Demo
本文的代碼根據(jù)之前DeepFM的代碼進(jìn)行改進(jìn),我們只介紹模型的實(shí)現(xiàn)部分,其他數(shù)據(jù)處理的細(xì)節(jié)大家可以參考我的github上的代碼.
模型輸入
模型的輸入主要有下面幾個(gè)部分:
self.feat_index = tf.placeholder(tf.int32, shape=[None,None], name='feat_index') self.feat_value = tf.placeholder(tf.float32, shape=[None,None], name='feat_value') self.label = tf.placeholder(tf.float32,shape=[None,1],name='label') self.dropout_keep_deep = tf.placeholder(tf.float32,shape=[None],name='dropout_deep_deep')
feat_index是特征的一個(gè)序號(hào),主要用于通過embedding_lookup選擇我們的embedding。feat_value是對(duì)應(yīng)的特征值,如果是離散特征的話,就是1,如果不是離散特征的話,就保留原來的特征值。label是實(shí)際值。還定義了dropout來防止過擬合。
權(quán)重構(gòu)建
權(quán)重主要分以下幾部分,偏置項(xiàng),一次項(xiàng)權(quán)重,embeddings,以及DNN的權(quán)重
def _initialize_weights(self): weights = dict() #embeddings weights['feature_embeddings'] = tf.Variable( tf.random_normal([self.feature_size,self.embedding_size],0.0,0.01), name='feature_embeddings') weights['feature_bias'] = tf.Variable(tf.random_normal([self.feature_size,1],0.0,1.0),name='feature_bias') weights['bias'] = tf.Variable(tf.constant(0.1),name='bias') #deep layers num_layer = len(self.deep_layers) input_size = self.embedding_size glorot = np.sqrt(2.0/(input_size + self.deep_layers[0])) weights['layer_0'] = tf.Variable( np.random.normal(loc=0,scale=glorot,size=(input_size,self.deep_layers[0])),dtype=np.float32 ) weights['bias_0'] = tf.Variable( np.random.normal(loc=0,scale=glorot,size=(1,self.deep_layers[0])),dtype=np.float32 ) for i in range(1,num_layer): glorot = np.sqrt(2.0 / (self.deep_layers[i - 1] + self.deep_layers[i])) weights["layer_%d" % i] = tf.Variable( np.random.normal(loc=0, scale=glorot, size=(self.deep_layers[i - 1], self.deep_layers[i])), dtype=np.float32) # layers[i-1] * layers[i] weights["bias_%d" % i] = tf.Variable( np.random.normal(loc=0, scale=glorot, size=(1, self.deep_layers[i])), dtype=np.float32) # 1 * layer[i] return weights
Embedding Layer這個(gè)部分很簡單啦,是根據(jù)feat_index選擇對(duì)應(yīng)的weights['feature_embeddings']中的embedding值,然后再與對(duì)應(yīng)的feat_value相乘就可以了:
# Embeddings self.embeddings = tf.nn.embedding_lookup(self.weights['feature_embeddings'],self.feat_index) # N * F * K feat_value = tf.reshape(self.feat_value,shape=[-1,self.field_size,1]) self.embeddings = tf.multiply(self.embeddings,feat_value) # N * F * K
Bi-Interaction Layer我們直接根據(jù)化簡后的結(jié)果進(jìn)行計(jì)算,得到一個(gè)K維的向量:
# sum-square-part self.summed_features_emb = tf.reduce_sum(self.embeddings, 1) # None * k self.summed_features_emb_square = tf.square(self.summed_features_emb) # None * K # squre-sum-part self.squared_features_emb = tf.square(self.embeddings) self.squared_sum_features_emb = tf.reduce_sum(self.squared_features_emb, 1) # None * K # second order self.y_second_order = 0.5 * tf.subtract(self.summed_features_emb_square, self.squared_sum_features_emb)
Deep Part將Bi-Interaction Layer層得到的結(jié)果經(jīng)過一個(gè)多層的神經(jīng)網(wǎng)絡(luò),得到交互項(xiàng)的輸出:
self.y_deep = self.y_second_order for i in range(0, len(self.deep_layers)): self.y_deep = tf.add(tf.matmul(self.y_deep, self.weights["layer_%d" % i]), self.weights["bias_%d" % i]) self.y_deep = self.deep_layers_activation(self.y_deep) self.y_deep = tf.nn.dropout(self.y_deep, self.dropout_keep_deep[i + 1])
得到預(yù)測輸出為了得到預(yù)測輸出,我們還需要兩部分,分別是偏置項(xiàng)和一次項(xiàng):
# first order term self.y_first_order = tf.nn.embedding_lookup(self.weights['feature_bias'], self.feat_index) self.y_first_order = tf.reduce_sum(tf.multiply(self.y_first_order, feat_value), 2) # bias self.y_bias = self.weights['bias'] * tf.ones_like(self.label)
而我們的最終輸出如下:
# out self.out = tf.add_n([tf.reduce_sum(self.y_first_order,axis=1,keep_dims=True), tf.reduce_sum(self.y_deep,axis=1,keep_dims=True), self.y_bias])
剩下的代碼就不介紹啦!好啦,本文只是提供一個(gè)引子,有關(guān)NFM的知識(shí)大家可以更多的進(jìn)行學(xué)習(xí)呦。
4、小結(jié)
NFM模型將FM與神經(jīng)網(wǎng)絡(luò)結(jié)合以提升FM捕捉特征間多階交互信息的能力。根據(jù)論文中實(shí)驗(yàn)結(jié)果,NFM的預(yù)測準(zhǔn)確度相較FM有明顯提升,并且與現(xiàn)有的并行神經(jīng)網(wǎng)絡(luò)模型相比,復(fù)雜度更低。
NFM本質(zhì)上還是基于FM,F(xiàn)M會(huì)讓一個(gè)特征固定一個(gè)特定的向量,當(dāng)這個(gè)特征與其他特征做交叉時(shí),都是用同樣的向量去做計(jì)算。這個(gè)是很不合理的,因?yàn)椴煌奶卣髦g的交叉,重要程度是不一樣的。因此,學(xué)者們提出了AFM模型(Attentional factorization machines),將attention機(jī)制加入到我們的模型中,關(guān)于AFM的知識(shí),我們下一篇來一探究竟。
-
神經(jīng)網(wǎng)絡(luò)
+關(guān)注
關(guān)注
42文章
4773瀏覽量
100890 -
dnn
+關(guān)注
關(guān)注
0文章
60瀏覽量
9058
原文標(biāo)題:推薦系統(tǒng)遇上深度學(xué)習(xí)(七)--NFM模型理論和實(shí)踐
文章出處:【微信號(hào):AI_shequ,微信公眾號(hào):人工智能愛好者社區(qū)】歡迎添加關(guān)注!文章轉(zhuǎn)載請注明出處。
發(fā)布評(píng)論請先 登錄
相關(guān)推薦
【我是電子發(fā)燒友】如何加速DNN運(yùn)算?
神經(jīng)網(wǎng)絡(luò)DNN知識(shí)點(diǎn)總結(jié)
什么是深度學(xué)習(xí)?使用FPGA進(jìn)行深度學(xué)習(xí)的好處?
什么是DNN_如何使用硬件加速DNN運(yùn)算
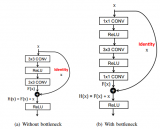
FM和FFM原理的探索和應(yīng)用的經(jīng)驗(yàn)
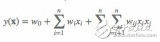
詳解DNN訓(xùn)練中出現(xiàn)的問題與解決方法方法
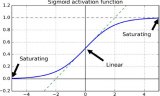
用于理解深度神經(jīng)網(wǎng)絡(luò)的CLass增強(qiáng)型注意響應(yīng)(CLEAR)方法
回顧3年來的所有主流深度學(xué)習(xí)CTR模型
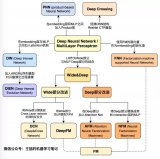
使用神經(jīng)網(wǎng)絡(luò)實(shí)現(xiàn)語音驅(qū)動(dòng)發(fā)音器官運(yùn)動(dòng)合成方法詳細(xì)資料說明
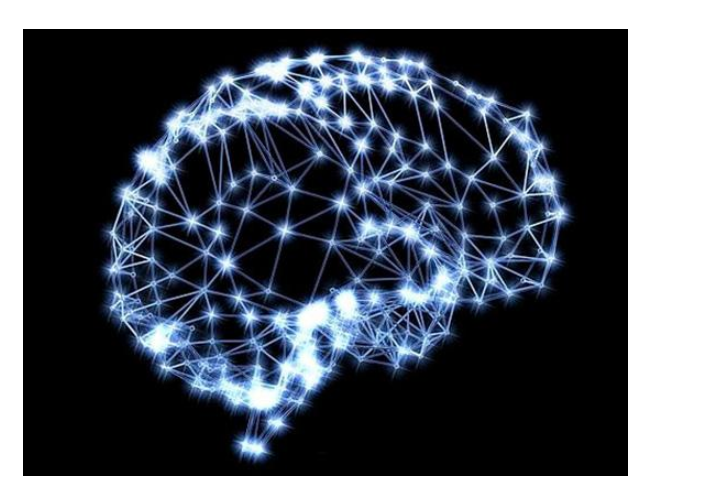
基于注意力機(jī)制的深度興趣網(wǎng)絡(luò)點(diǎn)擊率模型
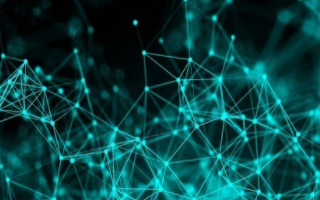
綜述深度神經(jīng)網(wǎng)絡(luò)的解釋方法及發(fā)展趨勢
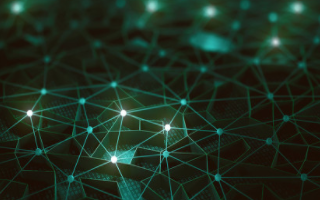
淺析深度神經(jīng)網(wǎng)絡(luò)(DNN)反向傳播算法(BP)

評(píng)論