異常檢測技術已經被應用于確保解決網絡安全和網聯車輛安全性等挑戰性問題。本文提出了這個領域的先前研究的分類。本文提出的分類學有3個總體維度,包括9個類別和38個子類別。從調查中得出的主要觀察結果是:真實世界的數據集很少被使用,大多數結果來自仿真;V2V / V2I通信和車載通信不一起考慮;提議的技術很少針對基線進行評估;網聯車輛的安全沒有網絡安全那么受關注。
I.介紹
Velosa等人 [1]預測到2020年將有25億輛網聯車輛上路。車聯網是指能夠連接到網絡的車輛,即它可以與其他車輛(V2V)或基礎設施(V2I)進行通信,因而可以增強信息娛樂功能以及可以減少碰撞和避免擁塞[2]復雜的等進行復雜功能的應用。在V2V的情況下,通常建議車輛之間形成車輛自組織網絡( VANET )。
雖然網聯車輛可以增加乘客的便利性以及行駛的安全性,但它們也很容易受到網絡攻擊[3]。一些研究[4]、[5]、[6]已經發現并證明了普通車輛中可利用的漏洞。網聯車輛連接數量的增加會增加這些漏洞的脆弱性的影響和普遍程度。此外,確保網聯車輛的安全性變得至關重要,因此各國政府加大了實現功能性VANET的力度[7]。
本文對自2000年初以來的研究進行了調查和分析,即對網聯車輛的安全和網絡安全問題進行異常檢測。異常檢測是識別不遵循預期模式[ 8 ]的數據點或事件的過程。據悉,這是第一項在此背景下調查異常檢測使用的研究。我們提出了一個基于3個總體類別和9個子類別的分類法。我們還有38個維度來分類所有的調查論文。
我們調查和分析后得出以下推論:
1)大多數研究(65篇調查論文中有37篇)是在仿真數據集上進行的(65篇調查論文中只有19篇使用了真實世界的數據集)。
2)V2X和車載通信基本上沒有被一起探索(65個調查論文中的1個除外),這使得研究比較分散。
3)與網絡安全相比,網聯車輛的安全性(65份調查論文中只有21份)的研究較少。
4)與基線相比,新提出的采用異常檢測技術的方法很少(在65份調查論文中只有4篇),導致我們提出的方法的有效性量化較差。
因此,我們建議,應該更加重視建立基準和基線技術,以此來評估新技術。此外,我們還主張更多地利用真實世界的數據,而不是仿真數據。
論文的其余部分組織如下:我們在第II部分和第III部分探討相關工作和我們的調查方法,并在第Ⅳ部分提出我們的分類法。
II.相關工作
與當前的調查不同,自20世紀初以來,以前的調查分別考慮了VANET和車載網絡。例如,outernali等人[ 9 ]調查了VANETs以及Sakiz等人提出的各種入侵檢測方法。[10]全面調查了所有可能的攻擊,并提出了與VANETs相關的檢測機制。這兩項研究都沒有考慮可能的基于車載網絡的網絡安全問題。很少有研究調查車載網絡中可能存在的威脅和對策。例如Liu等人、McCune等人和Kelberger等人 [11],[12],[13]提出了車載(控制器局域網(CAN),本地互連網絡(LIN),FlexRay等)的各種威脅和可能的對策,網絡安全問題(基于VANET的問題不是考慮)。我們目前的調查是第一次在網聯車輛的背景下審查異常檢測技術。
III.調查方法
為了確保可重復性,我們的調查遵循Wholin的滾雪球方法[14]如下。
范圍定義:繼Chandola等人之后。 [8]我們將異常檢測定義為“在不符合預期行為的數據中查找模式”。形成或學習模型,然后監測數據的一致性。
收集初步研究:我們在Google學術中通過關鍵字搜索確定了一組初始論文。我們這樣做是為了避免出版商的偏見。使用的關鍵詞是: "異常檢測"、"連接車輛"、" VANET "、" V2I "、" V2V "、"入侵檢測"、"不當行為檢測"、" CAN總線"、"車內"、"安全"、"安保"。
滾雪球:通過關鍵詞搜索收集的初始集可能并不詳盡。因此,我們進行了前后滾雪球式的收集,以收集初始集引用的所有參考文獻。然后通過閱讀摘要包括或排除論文,然后考慮范圍的徹底閱讀(從而消除超出我們范圍的論文)。這個滾雪球過程被重復進行,直到沒有新的相關論文被添加。我們最終研究了有65篇論文。
雖然Wholin [14]建議聯系該領域的知名研究人員以獲取更多相關文獻,但我們忽略了這一步驟,因為鑒于該領域的多樣性,我們無法最終確定最重要的研究人員。
數據提取和分類發展:通過Wholin的調查方法[14],一旦詳盡地收集了所有相關論文,我們就記下了每篇論文的關鍵要素。我們使用了一種開放式卡片分類技術[15],收集了關鍵點,以得出我們提議的分類的維度。開放式卡片分類技術是通過參與者之間的共識將關鍵元素組織成概念組的過程。我們使用這種技術來達到我們的38個維度(底層)。然后,我們使用自下而上的方法,將這些維度分為9個子類別(中級),我們后來根據排序的多次迭代將其歸入3個總體類別(頂級)。我們只在這項研究的作者中進行了公開卡片分類的過程,盡管這個過程通常涉及更大的群體[ 15 ]。最后,我們通過標記將收集到的每篇論文都歸入我們的分類體系,通過用它們占據的每個指定維度標記每個論文。
IV.分類
上述過程產生了一個分類法(見圖1),包含3個頂級類別,9個子類別和38個維度。這些類別代表了研究領域的更高層次特征。我們的目標是確定每篇論文所針對的威脅、解決方案和研究特征。特別是,抓住了研究特點,對實驗的類型和嚴謹性進行了闡述。
反過來,每個提議的類別包括幾個子類別。威脅特征有兩個子類別,解決方案特征有4個子類別,研究特征各有3個子類別,可以更好地捕捉每個類別的特征。子類別還具有如圖1所示的尺寸,這些尺寸捕捉并突出了區分每個子類別的技術差異,如下所示:
A.威脅特征
這一類別涉及每篇調查論文所涉及的威脅。我們將其劃分為兩個正交的子類別,如下所示。
1)攻擊面:攻擊面識別網聯車輛中的潛在弱點。例如,一篇論文可能是針對基于CAN總線的攻擊(其他總線由于缺乏足夠的文獻而未被考慮),而其他一些論文則專門關注偽造車輛ECU(電子控制單元)輸出的攻擊。
2)攻擊方法:一篇論文可能涉及不止一種攻擊方法。我們特別提請注意此子類別中的黑/灰/蟲洞攻擊維度。這些是基于路由的攻擊,包括丟棄、選擇性轉發或惡意重新路由VANET[16]中的通信數據包。
B.解決方案特征
這一類別代表了為應對威脅而提議的解決方案的性質。
1)動機:異常檢測技術是用于檢測威脅還是也提供對威脅的響應?
2)部署點:網聯車輛的哪一部分是建議部署的解決方案。例如,可以在車輛的ECU中或在VANET的中央管理機構(CA)或路側單元(RSU)中部署解決方案。
3)安全目標:是否保護了信息安全(完整性,機密性,可用性)[17]和/或網聯車輛的安全性。人身安全不在這項工作的范圍之內。
4)異常檢測方法:異常可以用多種方式檢測。分類法區分了所使用的異常檢測方法。我們在這里提請注意基于規則的方法維度,它只代表從車輛操作中自動推斷規則的研究,而不是那些從專家那里引出規則的研究。
C.研究特點
雖然上述類別根據已解決的威脅和已確定的維度區分了先前的研究,但這一類別涉及研究方法和數據。
1)科學性:該子類別可記錄一篇論文是屬于理論論文,實驗論文,實證論文還是調查論文。論文可以是類型的組合。
2)數據來源:該子類別記錄論文是否使用現實數據或仿真數據。
3)數據集:該子類別記錄論文是使用VANET還是車載(CAN總線等)或自然數據[18]。如果一篇論文觀察了運行中的連接車輛,并觀察了其自然運行環境中的數據,而沒有引入任何人工數據(例如,攻擊),則認為該論文使用了自然數據。
圖1.描述所有維度、類別、子類別的分類結構。框中的尺寸首字母縮略詞是表I的列標題
V.從分類法中得出的推論
表I列出了根據第IV節中描述的分類法對第III部分收集的論文進行的分類。以這種方式呈現論文分類的主要目的是從中得出推論和見解,在本文中,我們得出的推論側重于分析研究現狀。表I中的每篇論文在“PAP”欄中用第一個字母和最后一個字母表示第一作者的姓氏和出版年份。
A.關注
我們對調查的分析中發現了兩個問題。研究VANET的論文都沒有使用真實世界的數據。在我們調查和分類的所有論文中,VANET中甚至沒有一篇論文(在研究VANET數據的35/65篇論文中)使用真實數據(所有研究都是在仿真數據集上進行的(37/65篇論文))。我們可以從表I中看到這一點,觀察到在研究特征類別中沒有VANET和真實世界維度上都有標記的論文。總體而言,在65篇被調查的論文中,只有28篇使用真實世界的數據集(大部分在車載網絡研究中)。
我們所提出的基于異常檢測的解決方案很少針對基線進行評估。從表I中的經驗維度(EMP)可以看出,只有4篇被調查的論文(65篇調查論文中有4篇)評估了針對基線的擬議解決方案。我們預計建立一個量化改進的基線將是一個領域成熟的可喜跡象[79]。
B.差距
這個研究領域還有三個明顯的空白有待填補。
除了65篇調查論文中的1篇論文(Levi等[47])外,沒有其他論文通過同時分析車載和VANET數據來考慮保護網聯車輛:即使來自VANET或車載網絡的故障/惡意通信也可能影響網聯車輛的安全性。例如,如果網聯車輛的車載網絡受到危害,則可能開始將故障數據發送到VANET,而VANET又可能危及其他車輛。
與確保網絡安全相比,車聯網安全性受到的關注較少。在許多情況下,安全漏洞可能危及網聯車輛的安全性,反之亦然。因此,即使在沒有安全問題的情況下也確保網聯車輛的安全性是重要的。只有少數被調查的論文(65篇被調查論文中的13篇)也考慮應對已發現的威脅。大多數論文(65篇調查論文中的59篇)僅用于在異常檢測的幫助下監測威脅。
表Ⅰ
顯示分類論文分配的矩陣
VI.結論
為了提高連接車輛的安全性,本文對異常檢測進行了研究。異常檢測研究的廣泛使用和零散的發表導致了大量文獻的出現,其中存在許多空白和問題。為了找出這些差距和關注點,并對研究領域做了一個全面的了解,我們對65份相關論文進行了調查,在調查期間開發了一種新的分類法,并根據分類法對調查的論文進行了分類,以找出差距。
該調查表明:
大部分研究是在仿真數據上進行的(65份調查論文中有37篇);車載網絡數據和VANET數據很少被一起考慮來用于保護網聯車輛(65份調查論文中除1份外);
網聯車輛安全研究沒有得到網絡安全研究那么多關注(在65篇調查論文中只有13篇);
而且,許多研究沒有對照基線評估提出新的技術(在65篇調查論文中只有4篇這樣做),這可能導致難以量化的結果。
因此,我們敦促研究人員解決這些發現的問題,并定期用我們提議的分類學來分析研究,以了解研究的現狀及其演變。
REFERENCES
[1] A. Velosa, J. Hines, H. LeHong, and E.Perkins, “Predicts 2015: TheInternet of Things,” Gartner: Stamford, CT, USA,2014.
[2] C. Evans-Pughe, “The connected car,” IEEReview, vol. 51, no. 1, pp.42–46, 2005.
[3] P. Papadimitratos, L. Buttyan, T. Holczer,E. Schoch, J. Freudiger,M. Raya, Z. Ma, F. Kargl, A. Kung, and J.-P. Hubaux,“Securevehicular communication systems: design and architecture,” IEEE
Communications Magazine, vol. 46, no. 11,2008.
[4] S. Checkoway, D. McCoy, B. Kantor, D.Anderson, H. Shacham,S. Savage, K. Koscher, A. Czeskis, F. Roesner, T. Kohno etal.,“Comprehensive experimental analyses of automotive attack surfaces.”
in USENIX Security Symposium. SanFrancisco, 2011.
[5] C. Miller and C. Valasek, “Adventuresin automotive networks andcontrol units,” DEF CON, vol. 21, pp. 260–264, 2013.
[6] R. M. Ishtiaq Roufa, H. Mustafaa, S. O.Travis Taylora, W. Xua,M. Gruteserb, W. Trappeb, and I. Seskarb, “Security andprivacy vulnerabilities of in-car wireless networks: A tire pressure monitoringsystem case study,” in 19th USENIX Security Symposium, Washington DC, 2010, pp.11–13.
[7] U. DoT, “Connected vehicle pilotdeployment program,” 2016. [Online]. Available:https://www.its.dot.gov/pilots/index.htm
[8] V. Chandola, A. Banerjee, and V. Kumar,“Anomaly detection: A survey,” ACM computing surveys (CSUR), vol. 41, no. 3, p.15, 2009.
[9] M. Erritali and B. E. Ouahidi, “Areview and classification of various VANET intrusion detection systems,” in2013 National Security Days (JNS3), 2013, pp. 1–6.
[10] F. Sakiz and S. Sen, “A survey of attacksand detection mechanisms on intelligent transportation systems: VANETs andIoV,” Ad Hoc Networks, vol. 61, pp. 33–50, 2017.
[11] J. Liu, S. Zhang, W. Sun, and Y. Shi, “In-vehiclenetwork attacks and countermeasures: challenges and future directions,” IEEENetwork, vol. 31, no. 5, pp. 50–58, 2017.
[12] S. Abbott-McCune and L. A. Shay,“Intrusion prevention system of automotive network CAN bus,” in 2016 IEEEInternational Carnahan Conference on Security Technology (ICCST), 2016, pp.1–8.
[13] P. Kleberger, T. Olovsson, and E. Jonsson,“Security aspects of the in-vehicle network in the connected car,” in 2011 IEEEIntelligent Vehicles Symposium (IV), 2011, pp. 528–533.
[14] C. Wohlin, “Guidelines for snowballingin systematic literature studies and a replication in software engineering,” inProc. 18th Intl. Conf. on Evaluation and Assessment in Software Engineering,2014, p. 38.
[15] M. Soegaard and R. F. Dam, Theencyclopedia of human-computer interaction. The Interaction Design Foundation,2012, ch. 2.2: Card Sorting.
[16] V. S. Abel, “Survey of attacks on mobileadhoc wireless networks,” International Journal on Computer Science andEngineering, vol. 3, no. 2, pp. 826–829, 2011.
[17] J. Andress, The basics of informationsecurity: understanding the fundamentals of InfoSec in theory and practice.Syngress, 2014.
[18] T. A. Dingus, S. G. Klauer, V. L.Neale, A. Petersen, S. E. Lee, J. Sudweeks, M. Perez, J. Hankey, D. Ramsey, S.Gupta et al., “The 100-car naturalistic driving study, phase II-results of the100-car field experiment,” U.S DoT, Tech. Rep., 2006.
[19] K. M. Alheeti, L. Al-Jobouri, and K. McDonald-Maier,“Increasing the rate of intrusion detection based on a hybrid technique,” in2013 5th Computer Science and Electronic Engineering Conference(CEEC). IEEE, 2013, pp. 179–182.
[20] K. M. Alheeti, A. Gruebler, and K.McDonald-Maier, “An intrusion detection system against malicious attacks on thecommunication network of driverless cars,” in 2015 12th Annual IEEE Consumer Communicationsand Networking Conf. (CCNC), 2015, pp. 916–921.
[21] K. M. Alheeti, A. Gruebler, and K. McDonald-Maier,“On the detection of grey hole and rushing attacks in self-driving vehicular networks,”in 2015 7th Computer Science and Electronic Engineering Conference (CEEC).IEEE, 2015, pp. 231–236.
[22] K. M. Alheeti, A. Gruebler, and K.McDonald-Maier, “An intrusion detection system against black hole attacks onthe communication network of self-driving cars,” in 2015 6th InternationalConference on Emerging Security Technologies (EST). IEEE, 2015, pp. 86–91.
[23] K. M. Alheeti and K. McDonald-Maier,“Hybrid intrusion detection in connected self-driving vehicles,” in 22nd IEEEInternational Conference on Automation and Computing (ICAC), 2016, pp. 456–461.
[24] K. M. Alheeti and K. McDonald-Maier,“An intelligent intrusion detection scheme for self-driving vehicles based onmagnetometer sensors,” in 2016 International Conference for Students on AppliedEngineering (ICSAE). IEEE, 2016, pp. 75–78.
[25] K. M. Alheeti, A. Gruebler, K.McDonald-Maier, and A. Fernando, “Prediction of DoS attacks in external communication for self-drivingvehicles using a fuzzy Petri net model,” in 2016 IEEE International Conferenceon Consumer Electronics (ICCE), 2016, pp. 502–503.
[26] K. M. Alheeti, A. Gruebler, and K.McDonald-Maier, “Intelligent intrusion detection of grey hole and rushingattacks in self-driving vehicular networks,” Computers, vol. 5, no. 3, p. 16,2016.
[27] K. M. Alheeti, R. Al-Zaidi, J. Woods,and K. McDonald-Maier, “An intrusion detection scheme for driverless vehiclesbased gyroscope sensor profiling,” in 2017 IEEE International Conference onConsumer Electronics (ICCE), 2017, pp. 448–449.
[28] K. M. Alheeti, A. Gruebler, and K.McDonald-Maier, “Using discriminant analysis to detect intrusions in externalcommunication for self-driving vehicles,” Digital Communications and Networks,vol. 3, no. 3, pp. 180–187, 2017.
[29] M. Al-Mutaz, L. Malott, and S. Chellappan,“Detecting Sybil attacks in vehicular networks,” Journal of Trust Management,vol. 1, p. 4, 2014.
[30] K. C. Abdelaziz, N. Lagraa, and A.Lakas, “Trust model with delayed verification for message relay in VANETs,” in2014 International Wireless Communications and Mobile Computing Conference (IWCMC).IEEE, 2014, pp. 700–705.
[31] O. Berlin, A. Held, M. Matousek, and F.Kargl, “POSTER: Anomalybased misbehaviour detection in connected car backends,”in 2016 IEEE Vehicular Networking Conference (VNC), 2016, pp. 1–2.
[32] A. R. Beukman, G. P. Hancke, and B. J.Silva, “A multi-sensor system for detection of driver fatigue,” in 14thInternational Conference on Industrial Informatics (INDIN). IEEE, 2016, pp.870–873.
[33] N. Bissmeyer, “Misbehavior detectionand attacker identification in vehicular ad-hoc networks,” PhD Thesis, TUBerlin, 2014.
[34] N. Dutta and S. Chellappan, “A time-seriesclustering approach for Sybil attack detection in vehicular ad hoc networks,”in Proc. Intl. Conf. on Advances in Vehicular Systems, Technologies, andApplications, Nice, 2013, pp. 21–26.
[35] S. Dietzel, R. van der Heijden, H. Decke,and F. Kargl, “A flexible, subjective logic-based framework for misbehaviordetection in V2V networks,” in Proceeding of IEEE International Symposium on aWorld of Wireless, Mobile and Multimedia Networks 2014, 2014, pp. 1–6.
[36] P. Golle, D. Greene, and J. Staddon,“Detecting and correcting malicious data in VANETs,” in Proceedings of the 1stACM International Workshop on Vehicular Ad Hoc Networks. ACM, 2004, pp. 29–37.
[37] M. Ghosh, A. Varghese, A. Gupta, A. A.Kherani, and S. N. Muthaiah, “Detecting misbehaviors in VANET with integrated root-cause analysis,”Ad Hoc Networks, vol. 8, no. 7, pp. 778–790, 2010.
[38] M. Gmiden, M. H. Gmiden, and H.Trabelsi, “An intrusion detection method for securing in-vehicle CAN bus,” in2016 17th International Conference on Sciences and Techniques of AutomaticControl and Computer Engineering (STA). IEEE, 2016, pp. 176–180.
[39] A. Ganesan, J. Rao, and K. Shin,“Exploiting consistency among heterogeneous sensors for vehicle anomalydetection,” SAE, Tech. Rep. 2017-01-1654, 2017.
[40] T. Hoppe, S. Kiltz, and J. Dittmann,“Applying intrusion detection to automotive it-early insights and remainingchallenges,” vol. 4, pp. 226–235, Jan. 2009.
[41] R. E. Haas, D. P. F. Mller, P. Bansal,R. Ghosh, and S. S. Bhat, “Intrusion detection in connected cars,” in 2017 IEEE Intl. Conf. on ElectroInformation Technology (EIT), 2017, pp. 516–519.
[42] M.-J. Kang and J.-W. Kang, “Intrusiondetection system using deep neural network for in-vehicle network security,”PLoS ONE, vol. 11, no. 6, 2016.
[43] B. Khorashadi, F. Liu, D. Ghosal, M.Zhang, and C. N. Chuah, “Distributed automated incident detection with VGRID,”IEEE Wireless Communications, vol. 18, no. 1, pp. 64–73, 2011.
[44] N. Kumar and N. Chilamkurti, “Collaborativetrust aware intelligent intrusion detection in VANETs,” Computers &Electrical Engineering, vol. 40, no. 6, pp. 1981–1996, 2014.
[45] C. Ling and D. Feng, “An algorithm fordetection of malicious messages on CAN buses,” in 2012 National Conference onInformation Technology and Computer Science. Atlantis Press, 2012.
[46] X. Li, Z. Li, J. Han, and J. G. Lee,“Temporal outlier detection in vehicle traffic data,” in 2009 IEEE 25thInternational Conference on Data Engineering, 2009, pp. 1319–1322.
[47] M. Levi, Y. Allouche, and A. Kontorovich,“Advanced analytics for connected cars cyber security,” arXiv:1711.01939 [cs],2017.
[48] T. Leinm¨uller, A. Held, G. Sch¨afer,and A. Wolisz, “Intrusion detection in VANETs,” in In Proceedings of 12th IEEEInternational Conference on Network Protocols (ICNP 2004) Student PosterSession, 2004.
[49] T. Leinm¨uller, E. Schoch, and C.Maihofer, “Security requirements and solution concepts in vehicular ad hoc networks,”in 4th IEEE Conf. on Wireless on Demand Network Systems and Services, 2007, pp.84–91.
[50] M. Marchetti, D. Stabili, A. Guido,and M. Colajanni, “Evaluation of anomaly detection for in-vehicle networksthrough informationtheoretic algorithms,” in 2016 IEEE 2nd International Forumon Research and Technologies for Society and Industry Leveraging a BetterTomorrow (RTSI), 2016, pp. 1–6.
[51] M. Marchetti and D. Stabili, “Anomalydetection of CAN bus messages through analysis of ID sequences,” in IntelligentVehicles Symposium (IV), 2017 IEEE. IEEE, 2017, pp. 1577–1583.
[52] F. Martinelli, F. Mercaldo, V. Nardone,and A. Santone, “Car hacking identification through fuzzy logic algorithms,” in2017 IEEE International Conference on Fuzzy Systems (FUZZ-IEEE), 2017, pp. 1–7.
[53] M. M¨uter, A. Groll, and F. C. Freiling,“A structured approach to anomaly detection for in-vehicle networks,” in 20106th International Conference on Information Assurance and Security, 2010, pp.92–98.
[54] M. M¨uter and N. Asaj, “Entropy-based anomalydetection for invehicle networks,” in 2011 IEEE Intelligent Vehicles Symposium(IV), 2011, pp. 1110–1115.
[55] L. Maglaras, “A novel distributedintrusion detection system for vehicular ad hoc networks,” InternationalJournal of Advanced Computer Science and Applications(IJACSA), vol. 6, 2015.
[56] M. Markovitz and A. Wool, “Fieldclassification, modeling and anomaly detection in unknown CAN bus networks,”Vehicular Communications, vol. 9, pp. 43–52, 2017.
[57] S. N. Narayanan, S. Mittal, and A. Joshi,“Using data analytics to detect anomalous states in vehicles,” arXiv:1512.08048[cs], 2015.
[58] V. L. Praba and A. Ranichitra, “Detectingmalicious vehicles and regulating traffic in VANET using RAODV protocol,”International Journal of Computer Applications, vol. 84, no. 1, 2013.
[59] O. Pattnaik and B. K. Pattanayak,“Security in vehicular ad hoc network based on intrusion detection system,”American Journal of Applied Sciences, vol. 11, no. 2, pp. 337–346, 2014.
[60] M. Raya, P. Papadimitratos, I. Aad, D.Jungels, and J. p Hubaux, “Eviction of misbehaving and faulty nodes in vehicular networks,” IEEEJournal on Selected Areas in Communications, vol. 25, no. 8, pp. 1557–1568,2007.
[61] R. Rieke, M. Seidemann, E. K. Talla,D. Zelle, and B. Seeger, “Behavior analysis for safety and security inautomotive systems,” in 2017 25th Euromicro International Conference on Parallel,Distributed and Network-Based Processing (PDP), 2017, pp. 381–385.
[62] J. Rezgui and S. Cherkaoui, “Detectingfaulty and malicious vehicles using rule-based communications data mining,” in2011 IEEE 36th Conference on Local Computer Networks, 2011, pp.827–834.
[63] H. M. Song, H. R. Kim, and H. K. Kim,“Intrusion detection system based on the analysis of time intervals of CANmessages for invehicle network,” in 2016 International Conference onInformation Networking (ICOIN). IEEE, 2016, pp. 63–68.
[64] H. Sedjelmaci, T. Bouali, and S. M.Senouci, “Detection and prevention from misbehaving intruders in vehicularnetworks,” in 2014 IEEE Global Communications Conference, 2014, pp. 39–44.
[65] H. Sedjelmaci and S. M. Senouci, “A newintrusion detection framework for vehicular networks,” in 2014 IEEEInternational Conference on Communications (ICC), 2014, pp. 538–543.
[66] D. Tian, Y. Wang, G. Lu, and G. Yu, “Avehicular ad hoc networks intrusion detection system based on BUSNet,” in 20102nd International Conference on Future Computer and Communication, vol. 1. IEEE,2010, pp. V1–225–V1–229.
[67] A. Theissler, “Anomaly detection inrecordings from in-vehicle networks,” Big data and applications, p. 23, 2014.
[68] A. Taylor, N. Japkowicz, and S. Leblanc,“Frequency-based anomaly detection for the automotive CAN bus,” in 2015 IEEEWorld Congress on Industrial Control Systems Security (WCICSS), 2015, pp.45–49.
[69] A. Taylor, S. Leblanc, and N.Japkowicz, “Anomaly detection in automobile control network data with longshort-term memory networks,” in 2016 IEEE International Conference on DataScience and Advanced Analytics (DSAA), 2016, pp. 130–139.
[70] A. Theissler, “Detecting known andunknown faults in automotive systems using ensemble-based anomaly detection,”Knowledge-Based Systems, vol. 123, pp. 163–173, 2017.
[71] D. K. Vasistha, “Detecting anomaliesin controller area network for automobiles,” Ph.D. dissertation, Texas A&MUniversity, 2017.
[72] O. A. Wahab, A. Mourad, H. Otrok, andJ. Bentahar, “CEAP: SVMbased intelligent detection model for clusteredvehicular ad hoc networks,” Expert Systems with Applications, vol. 50, pp.40–54, 2016.
[73] B. Xiao, B. Yu, and C. Gao, “Detectionand localization of Sybil nodes in VANETs,” in Proceedings of the 2006 Workshopon Dependability Issues in Wireless Ad Hoc Networks and Sensor Networks. ACM, 2006,pp. 1–8.
[74] S. Yang, Z. Liu, J. Li, S. Wang, andF. Yang, “Anomaly detection for Internet of Vehicles: A trust management schemewith affinity propagation,” Mobile Information Systems, vol. 2016, pp. 1–10,2016.
[75] B. Yu, C.-Z. Xu, and B. Xiao, “DetectingSybil attacks in VANETs,” Journal of Parallel and Distributed Computing, vol.73, no. 6, pp. 746–756, 2013.
[76] Y. Zhang and G. Cao, “V-PADA: Vehicle-platoon-awaredata access in VANETs,” IEEE Transactions on Vehicular Technology, vol. 60, no.5, pp. 2326–2339, 2011.
[77] M. Zhang, C. Chen, T. Wo, T. Xie, M.Z. A. Bhuiyan, and X. Lin, “SafeDrive: online driving anomaly detection from large-scale vehicledata,” IEEE Transactions on Industrial Informatics, vol. 13, no. 4, pp. 2087–2096,2017.
[78] K. Zaidi, M. B. Milojevic, V.Rakocevic, A. Nallanathan, and M. Rajarajan, “Host-based intrusiondetection for vanets: A statistical approach to rogue node detection,” IEEETransactions on Vehicular Technology, vol. 65, no. 8, pp. 6703–6714, 2016.
[79]S. Lessmann, B. Baesens, C. Mues, and S. Pietsch, “Benchmarking classificationmodels for software defect prediction: A proposed framework and novelfindings,” IEEE Transactions on Software Engineering, vol. 34, no. 4, pp.485–496, 2008.
-
網絡安全
+關注
關注
10文章
3155瀏覽量
59701 -
V2X
+關注
關注
25文章
209瀏覽量
43513
原文標題:網聯車輛網絡安全異常檢測綜述 | 智車科技
文章出處:【微信號:IV_Technology,微信公眾號:智車科技】歡迎添加關注!文章轉載請注明出處。
發布評論請先 登錄
相關推薦
專家解讀 | NIST網絡安全框架(1):框架概覽
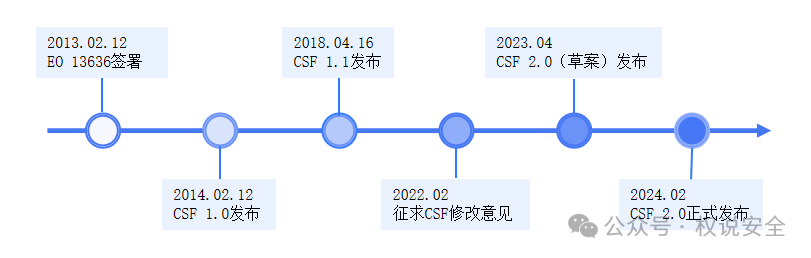
2020 年網絡安全的四大變化
為什么網絡攻擊無法與自動駕駛相匹敵
網絡安全類學習資源相關資料推薦
網絡安全檢測與監控技術的研究
探討2019年的網絡安全重要趨勢
入侵檢測技術在網絡安全中有什么樣的應用
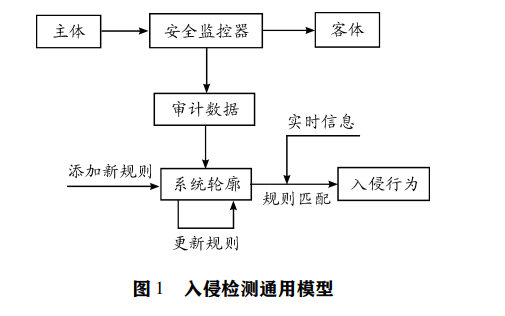
評論